Publications
2023
- S. Gravelle, S. Haber-Pohlmeier, C. Mattea, S. Stapf, C. Holm, and A. Schlaich, “NMR Investigation of Water in Salt Crusts: Insights from Experiments and Molecular Simulations,” Langmuir, vol. 39, no. 22, Art. no. 22, May 2023, doi: 10.1021/acs.langmuir.3c00036.
- Molpeceres, G., Zaverkin, V., Furuya, K., Aikawa, Y., and Kästner, J., “Reaction dynamics on amorphous solid water surfaces using interatomic machine-learned potentials - Microscopic energy partition revealed from the P + H → PH reaction,” Astronomy & Astrophysics, vol. 673, p. A51, 2023, doi: 10.1051/0004-6361/202346073.
- C. Lohrmann and C. Holm, “Optimal motility strategies for self-propelled agents to explore porous media,” Physical Review B, vol. 108, no. 5, Art. no. 5, Nov. 2023, doi: 10.1103/PhysRevE.108.054401.
- J. Wachlmayr, G. Fläschner, K. Pluhackova, W. Sandtner, C. Siligan, and A. Horner, “Entropic barrier of water permeation through single-file channels,” Communications Chemistry, vol. 6, no. 1, Art. no. 1, Jun. 2023, doi: 10.1038/s42004-023-00919-0.
- V. Artemov et al., “The Three-Phase Contact Potential Difference Modulates the Water Surface Charge,” The Journal of Physical Chemistry Letters, vol. 14, no. 20, Art. no. 20, May 2023, doi: 10.1021/acs.jpclett.3c00479.
- V. Zaverkin, D. Holzmüller, L. Bonfirraro, and J. Kästner, “Transfer learning for chemically accurate interatomic neural network potentials,” Physical Chemistry Chemical Physics, vol. 25, no. 7, Art. no. 7, 2023, doi: 10.1039/D2CP05793J.
- C. Lohrmann and C. Holm, “A novel model for biofilm initiation in porous media flow,” Soft Matter, vol. 19, no. 36, Art. no. 36, 2023, doi: 10.1039/D3SM00575E.
- M. Degen et al., “Structural basis of NINJ1-mediated plasma membrane rupture in cell death,” Nature, vol. 618, no. 7967, Art. no. 7967, Jun. 2023, doi: 10.1038/s41586-023-05991-z.
- J. Rettberg et al., “Port-Hamiltonian fluid–structure interaction modelling and structure-preserving model order reduction of a classical guitar,” Mathematical and Computer Modelling of Dynamical Systems, vol. 29, no. 1, Art. no. 1, 2023, doi: 10.1080/13873954.2023.2173238.
- H. Jäger, A. Schlaich, J. Yang, C. Lian, S. Kondrat, and C. Holm, “A screening of results on the decay length in concentrated electrolytes,” Faraday Discussions, Feb. 2023, doi: 10.1039/d3fd00043e.
- K. Gubaev, V. Zaverkin, P. Srinivasan, A. I. Duff, J. Kästner, and B. Grabowski, “Performance of two complementary machine-learned potentials in modelling chemically complex systems,” NPJ Computational Materials, vol. 9, p. 129, 2023, doi: 10.1038/s41524-023-01073-w.
2022
- K. Pluhackova, V. Schittny, P.-C. Bürkner, C. Siligan, and A. Horner, “Multiple pore lining residues modulate water permeability of GlpF,” Protein Science, vol. 31, no. 10, Art. no. 10, 2022, doi: https://doi.org/10.1002/pro.4431.
- M. Pechlaner, W. F. van Gunsteren, N. Hansen, and L. J. Smith, “Molecular dynamics simulation or structure refinement of proteins: are solvent molecules required? A case study using hen lysozyme,” European Biophysics Journal, vol. 51, no. 3, Art. no. 3, Apr. 2022, doi: 10.1007/s00249-022-01593-1.
- W. F. van Gunsteren, M. Pechlaner, L. J. Smith, B. Stankiewicz, and N. Hansen, “A Method to Derive Structural Information on Molecules from Residual Dipolar Coupling NMR Data,” The Journal of Physical Chemistry B, vol. 126, no. 21, Art. no. 21, May 2022, doi: 10.1021/acs.jpcb.2c02410.
- I. Tischler, F. Weik, R. Kaufmann, M. Kuron, R. Weeber, and C. Holm, “A thermalized electrokinetics model including stochastic reactions suitable for multiscale simulations of reaction-advection-diffusion systems,” Journal of Computational Science, vol. 63, p. 101770, 2022, doi: 10.1016/j.jocs.2022.101770.
- V. Korn and K. Pluhackova, “Not sorcery after all: Roles of multiple charged residues in membrane insertion of gasdermin-A3,” Frontiers in Cell and Developmental Biology, vol. 10, 2022, doi: 10.3389/fcell.2022.958957.
- N. E. R. Zimmermann, G. Guevara-Carrion, J. Vrabec, and N. Hansen, “Predicting and Rationalizing the Soret Coefficient of Binary Lennard-Jones Mixtures in the Liquid State,” Advanced Theory and Simulations, vol. 5, no. 11, Art. no. 11, Jul. 2022, doi: 10.1002/adts.202200311.
- S. Liese, A. Schlaich, and R. R. Netz, “Dielectric Constant of Aqueous Solutions of Proteins and Organic Polymers from Molecular Dynamics Simulations,” The Journal of Chemical Physics, 2022, doi: 10.1063/5.0089397.
- D. Markthaler, H. Kraus, and N. Hansen, “Binding free energies for the SAMPL8 CB8 ‘Drugs of Abuse’ challenge from umbrella sampling combined with Hamiltonian replica exchange,” Journal of Computer-Aided Molecular Design, vol. 36, pp. 1–9, 2022, doi: 10.1007/s10822-021-00439-w.
- M. Gültig, J. P. Range, B. Schmitz, and J. Pleiss, “Integration of Simulated and Experimentally Determined Thermophysical Properties of Aqueous Mixtures by ThermoML,” Journal of Chemical & Engineering Data, vol. 67, no. 11, Art. no. 11, 2022, doi: 10.1021/acs.jced.2c00391.
- D. Markthaler, M. Fleck, B. Stankiewicz, and N. Hansen, “Exploring the Effect of Enhanced Sampling on Protein Stability Prediction,” Journal of Chemical Theory and Computation, vol. 18, no. 4, Art. no. 4, Mar. 2022, doi: 10.1021/acs.jctc.1c01012.
- N. Gössweiner-Mohr et al., “The Hidden Intricacies of Aquaporins: Remarkable Details in a Common Structural Scaffold,” Small, vol. 18, no. 31, Art. no. 31, 2022, doi: https://doi.org/10.1002/smll.202202056.
- C. Kessler et al., “Influence of layer slipping on adsorption of light gases in covalent organic frameworks: A combined experimental and computational study,” Microporous and Mesoporous Materials, vol. 336, p. 111796, May 2022, doi: 10.1016/j.micromeso.2022.111796.
- V. Zaverkin, D. Holzmüller, R. Schuldt, and J. Kästner, “Predicting properties of periodic systems from cluster data: A case study of liquid water,” The Journal of Chemical Physics, vol. 156, no. 11, Art. no. 11, 2022, doi: 10.1063/5.0078983.
- V. Zaverkin, J. Netz, F. Zills, A. Köhn, and J. Kästner, “Thermally Averaged Magnetic Anisotropy Tensors via Machine Learning Based on Gaussian Moments,” Journal of Chemical Theory and Computation, vol. 18, pp. 1–12, 2022, doi: 10.1021/acs.jctc.1c00853.
2021
- L. J. Smith, W. F. van Gunsteren, B. Stankiewicz, and N. Hansen, “On the use of 3J-coupling NMR data to derive structural information on proteins,” Journal of Biomolecular NMR, vol. 75, no. 1, Art. no. 1, Jan. 2021, doi: 10.1007/s10858-020-00355-5.
- J. Zeman, S. Kondrat, and C. Holm, “Ionic screening in bulk and under confinement,” The Journal of Chemical Physics, vol. 155, no. 20, Art. no. 20, 2021, doi: 10.1063/5.0069340.
- A. Wagner et al., “Permeability Estimation of Regular Porous Structures: A Benchmark for Comparison of Methods,” Transport in Porous Media, vol. 138, no. 1, Art. no. 1, 2021, doi: 10.1007/s11242-021-01586-2.
- A. Schlaich, D. Jin, L. Bocquet, and B. Coasne, “Electronic screening using a virtual Thomas--Fermi fluid for predicting wetting and phase transitions of ionic liquids at metal surfaces,” Nature Materials, Nov. 2021, doi: 10.1038/s41563-021-01121-0.
- K. Szuttor, P. Kreissl, and C. Holm, “A numerical investigation of analyte size effects in nanopore sensing systems,” The Journal of Chemical Physics, vol. 155, no. 13, Art. no. 13, 2021, doi: 10.1063/5.0065085.
- D. Markthaler and N. Hansen, “Umbrella sampling and double decoupling data for methanol binding to Candida antarctica lipase B,” Data in Brief, vol. 39, p. 107618, Dec. 2021, doi: 10.1016/j.dib.2021.107618.
- D. Born and J. Kästner, “Geometry Optimization in Internal Coordinates Based on Gaussian Process Regression: Comparison of Two Approaches,” Journal of Chemical Theory and Computation, vol. 17, no. 9, Art. no. 9, 2021, doi: 10.1021/acs.jctc.1c00517.
- G. Molpeceres, V. Zaverkin, N. Watanabe, and J. Kästner, “Binding energies and sticking coefficients of H₂ on crystalline and amorphous CO ice,” Astronomy & Astrophysics, vol. 648, p. A84, 2021, doi: 10.1051/0004-6361/202040023.
- K. Szuttor, F. Weik, J.-N. Grad, and C. Holm, “Modeling the current modulation of bundled DNA structures in nanopores,” The Journal of Chemical Physics, vol. 154, no. 5, Art. no. 5, 2021, doi: 10.1063/5.0038530.
- J. M. Riede, C. Holm, S. Schmitt, and D. F. B. Haeufle, “The control effort to steer self-propelled microswimmers depends on their morphology: comparing symmetric spherical versus asymmetric L -shaped particles,” Royal Society Open Science, vol. 8, no. 9, Art. no. 9, Sep. 2021, doi: 10.1098/rsos.201839.
- L. J. Smith, W. F. Gunsteren, and N. Hansen, “On the Use of Side-Chain NMR Relaxation Data to Derive Structural and Dynamical Information on Proteins: A Case Study Using Hen Lysozyme,” ChemBioChem, vol. 22, no. 6, Art. no. 6, Dec. 2021, doi: 10.1002/cbic.202000674.
- M. Kuron, C. Stewart, J. de Graaf, and C. Holm, “An extensible lattice Boltzmann method for viscoelastic flows: complex and moving boundaries in Oldroyd-B fluids,” The European Physical Journal E, vol. 44, no. 1, Art. no. 1, 2021, doi: 10.1140/epje/s10189-020-00005-6.
2020
- S. Tovey et al., “DFT accurate interatomic potential for molten NaCl from machine learning,” The Journal of Physical Chemistry C, vol. 124, no. 47, Art. no. 47, 2020, doi: 10.1021/acs.jpcc.0c08870.
- G. Molpeceres, V. Zaverkin, and J. Kästner, “Neural-network assisted study of nitrogen atom dynamics on amorphous solid water – I. adsorption and desorption,” Mon. Not. R. Astron. Soc., vol. 499, pp. 1373–1384, 2020, doi: 10.1093/mnras/staa2891.
- N. Hansen et al., “A Suite of Advanced Tutorials for the GROMOS Biomolecular Simulation Software Article v1.0,” Living Journal of Computational Molecular Science, vol. 2, no. 1, Art. no. 1, 2020, doi: 10.33011/livecoms.2.1.18552.
- J. Zeman, S. Kondrat, and C. Holm, “Bulk ionic screening lengths from extremely large-scale molecular dynamics simulations,” Chemical Communications, vol. 56, no. 100, Art. no. 100, 2020, doi: 10.1039/D0CC05023G.
- J. Gebhardt, M. Kiesel, S. Riniker, and N. Hansen, “Combining Molecular Dynamics and Machine Learning to Predict Self-Solvation Free Energies and Limiting Activity Coefficients,” Journal of Chemical Information and Modeling, vol. 60, no. 11, Art. no. 11, Aug. 2020, doi: 10.1021/acs.jcim.0c00479.
- G. Sivaraman et al., “Machine-learned interatomic potentials by active learning: amorphous and liquid hafnium dioxide,” npj Computational Materials, vol. 6, no. 1, Art. no. 1, Jul. 2020, doi: 10.1038/s41524-020-00367-7.
- V. Zaverkin and J. Kästner, “Gaussian Moments as Physically Inspired Molecular Descriptors for Accurate and Scalable Machine Learning Potentials,” Journal of Chemical Theory and Computation, vol. 16, pp. 5410–5421, 2020, doi: 10.1021/acs.jctc.0c00347.
- K. Breitsprecher et al., “How to speed up ion transport in nanopores,” Nature Communications, vol. 11, no. 1, Art. no. 1, Nov. 2020, doi: 10.1038/s41467-020-19903-6.
- A. Denzel and J. Kästner, “Hessian Matrix Update Scheme for Transition State Search Based on Gaussian Process Regression,” Journal of Chemical Theory and Computation, vol. 16, no. 8, Art. no. 8, Jul. 2020, doi: 10.1021/acs.jctc.0c00348.
2019
- J. Zeman, C. Holm, and J. Smiatek, “The Effect of Small Organic Cosolutes on Water Structure and Dynamics,” Journal of Chemical & Engineering Data, vol. 65, no. 3, Art. no. 3, Aug. 2019, doi: 10.1021/acs.jced.9b00577.
- A. Denzel, B. Haasdonk, and J. Kästner, “Gaussian Process Regression for Minimum Energy Path Optimization and Transition State Search,” The Journal of Physical Chemistry A, vol. 123, no. 44, Art. no. 44, 2019, doi: 10.1021/acs.jpca.9b08239.
2018
- A. Denzel and J. Kästner, “Gaussian Process Regression for Transition State Search,” Journal of Chemical Theory and Computation, vol. 14, no. 11, Art. no. 11, 2018, doi: 10.1021/acs.jctc.8b00708.
- A. Denzel and J. Kästner, “Gaussian process regression for geometry optimization,” Journal of Chemical Physics, vol. 148, no. 9, Art. no. 9, 2018, doi: 10.1063/1.5017103.
Published software
Published data
2023
- J. Rettberg et al., “Replication Data for: Port-Hamiltonian Fluid-Structure Interaction Modeling and Structure-Preserving Model Order Reduction of a Classical Guitar.” 2023. doi: 10.18419/darus-3248.
Project Network Coordinators
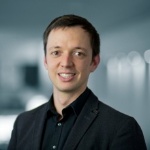
Felix Fritzen
Prof. Dr.-Ing. Dipl.-Math. techn.Data Analytics in Engineering
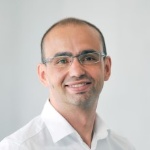
Blazej Grabowski
Prof. Dr. rer. nat.Materials Design
[Photo: (c) SimTech/Max Kovalenko]