So far, classical simulations and Machine Learning (ML) are separated fields based on different paradigms. This Project Network paves the way for integrating them with joint model-based and data-driven predictions capable of tackling simulation problems that are currently out of reach. In addition, opening the black box of ML through visualization will give non-experts access to these tools. On the one hand, the network will focus methodologically on supervised, unsupervised, and reinforcement learning. We will develop, analyze, and implement novel ML strategies adapted to the characteristics of simulation data, such as symmetries, invariances, and physical prior knowledge. And, surprisingly, even deterministic trajectories are incompatible with statistical assumptions required for standard learning guarantees for ML methods.
The new ML techniques will further support visual exploration and interactive control by users and will be applied to the different stages of the simulation cycle, specifically in the modeling, prediction, visualization, and visual analytics stages. On the other hand, and complementary to these methodological considerations, PN 6 will aim at broad collaborations with the other networks by disseminating our network’s ML and visualization competences as well as tools to the diverse application fields. To this end, we will combine ML expertise in mathematics and computer science in this network with domain expertise from collaborating networks.
RQ 2 Sim4ML: How can simulation data augment real-world/experimental data for ML?
RQ 3 VisML2: How can we use visualization to better understand and control ML and, vice versa, how can we leverage ML for data-integrated visualization?
Ongoing projects
PN 6-1 (II) | Greedy deep kernel methods for data-based-modelling in Biomechanics |
PN 6-2 (II) | Towards parameter-dependent data-enriched physics-informed machine learning |
PN 6-3 (II) | Gaussian process techniques for differential equations |
PN 6-4 (II) | Visual analytics for machine learning |
PN 6-5 (II) | Interpretable and explainable cognitive inspired machine learning systems |
PN 6-7 | Machine learning for Bayesian model building (M4LBMB) |
PN 6-8 | Visual data science to master complex simulation ensembles |
PN 6-9 | Analytical models combined with data-driven techniques in dynamic compton scattering tomography |
PN 6-10 | Generalization and robustness of learned simulators (GRLSim) |
PN 6-11 | Data-integrated simulation of interactive behaviour |
PN 6-13 | Science-constrained learning |
PN 6-14 | |
PN 6-15 |
Machine learning and reservoir computing with many-body dynamics
|
Completed projects
Ongoing associated projects
Completed associated projects
Project Network Coordinators
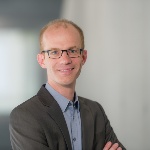
Dirk Pflüger
Prof. Dr. rer. nat.Scientific Computing | Vice-Head of GS SimTech
[Photo: SimTech/Max Kovalenko]
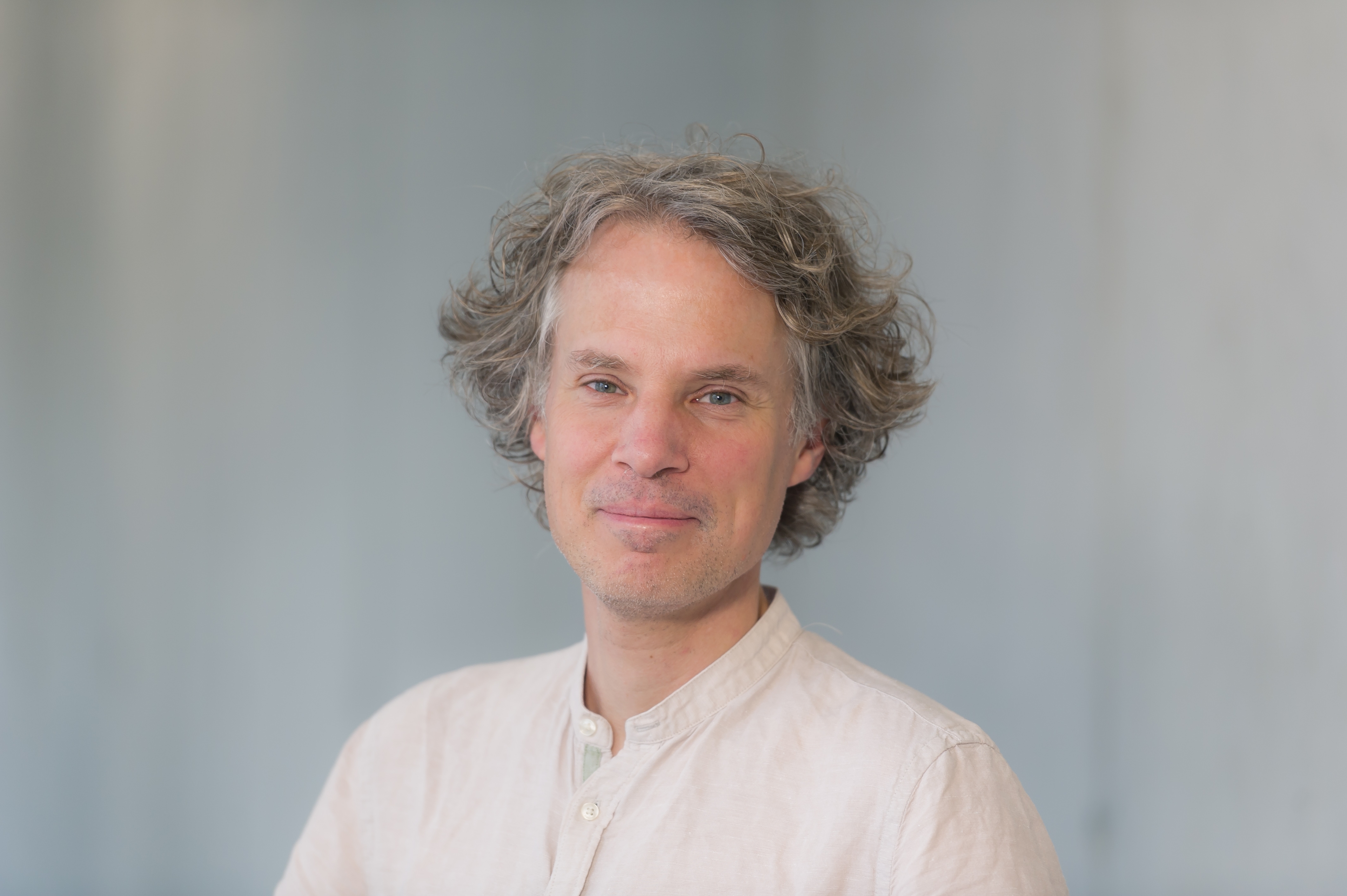
Ingo Steinwart
Univ.-Prof. Dr. rer. nat.Stochastics
[Photo: SimTech/Max Kovalenko]
Mathias Niepert
Prof. Dr.Machine Learning for Simulation Science