Publications PN 6
2024
- A. Penzkofer, L. Shi, and A. Bulling, “VSA4VQA: Scaling A Vector Symbolic Architecture To Visual Question Answering on Natural Images,” in Proc. 46th Annual Meeting of the Cognitive Science Society (CogSci), 2024.
- J. Elenter, L. F. O. Chamon, and A. Ribeiro, “Near-optimal solutions of constrained learning problems,” in International Conference on Learning Representations~(ICLR), 2024.
- M. Álvarez Chaves, H. V. Gupta, U. Ehret, and A. Guthke, “On the Accurate Estimation of Information-Theoretic Quantities from Multi-Dimensional Sample Data,” Entropy, vol. 26, Art. no. 5, 2024, doi: 10.3390/e26050387.
- T. Munz-Körner and D. Weiskopf, “Exploring visual quality of multidimensional time series projections,” Visual Informatics, 2024, doi: https://doi.org/10.1016/j.visinf.2024.04.004.
- F. Zills, M. R. Schäfer, N. Segreto, J. Kästner, C. Holm, and S. Tovey, “Collaboration on Machine-Learned Potentials with IPSuite: A Modular Framework for Learning-on-the-Fly,” The Journal of Physical Chemistry B, Apr. 2024, doi: 10.1021/acs.jpcb.3c07187.
- V. Zaverkin et al., “Uncertainty-biased molecular dynamics for learning uniformly accurate interatomic potentials,” npj Comput. Mater., vol. 10, p. 83, 2024, doi: 10.1038/s41524-024-01254-1.
2023
- D. Holzmüller, “Regression from linear models to neural networks: double descent, active learning, and sampling,” University of Stuttgart, 2023.
- J. Cervino, L. F. O. Chamon, B. D. Haeffele, R. Vidal, and A. Ribeiro, “Learning Globally Smooth Functions on Manifolds,” in International Conference on Machine Learning~(ICML), 2023.
- N. Schäfer et al., “Visual Analysis of Scene-Graph-Based Visual Question Answering,” in Proceedings of the 16th International Symposium on Visual Information Communication and Interaction, in VINCI ’23. Guangzhou, China: Association for Computing Machinery, Oct. 2023, pp. 1–8. doi: 10.1145/3615522.3615547.
- I. Hounie, L. F. O. Chamon, and A. Ribeiro, “Automatic Data Augmentation via Invariance-Constrained Learning,” in International Conference on Machine Learning~(ICML), 2023.
- D. Holzmüller, V. Zaverkin, J. Kästner, and I. Steinwart, “A Framework and Benchmark for Deep Batch Active Learning for Regression,” Journal of Machine Learning Research, vol. 24, Art. no. 164, 2023, [Online]. Available: http://jmlr.org/papers/v24/22-0937.html
- J. Rettberg et al., “Port-Hamiltonian fluid–structure interaction modelling and structure-preserving model order reduction of a classical guitar,” Mathematical and Computer Modelling of Dynamical Systems, vol. 29, Art. no. 1, 2023, doi: 10.1080/13873954.2023.2173238.
- R. Bauer et al., “Visual Ensemble Analysis of Fluid Flow in Porous Media Across Simulation Codes and Experiment,” Transport in Porous Media, 2023, doi: 10.1007/s11242-023-02019-y.
- J. Gödeke and G. Rigaud, “Imaging based on Compton scattering: model uncertainty and data-driven reconstruction methods,” Inverse Problems, vol. 39, Art. no. 3, Feb. 2023, doi: 10.1088/1361-6420/acb2ed.
- V. Zaverkin, D. Holzmüller, L. Bonfirraro, and J. Kästner, “Transfer learning for chemically accurate interatomic neural network potentials,” Physical Chemistry Chemical Physics, vol. 25, Art. no. 7, 2023, doi: 10.1039/D2CP05793J.
- L. Pfitzer, J. Heitkämper, J. Kästner, and R. Peters, “Use of the N–O Bonds in N-Mesyloxyamides and N-Mesyloxyimides To Gain Access to 5-Alkoxy-3,4-dialkyloxazol-2-ones and 3-Hetero-Substituted Succinimides: A Combined Experimental and Theoretical Study,” Synthesis, vol. 55, Art. no. 26, 2023, doi: 10.1055/s-0042-1751447.
- B. N. Hahn, G. Rigaud, and R. Schmähl, “A class of regularizations based on nonlinear isotropic diffusion for inverse problems,” IMA Journal of Numerical Analysis, Feb. 2023, doi: 10.1093/imanum/drad002.
- A. Straub, G. K. Karch, J. Steigerwald, F. Sadlo, B. Weigand, and T. Ertl, “Visual Analysis of Interface Deformation in Multiphase Flow,” Journal of Visualization, vol. 26, Art. no. 6, 2023, doi: 10.1007/s12650-023-00939-x.
- K. Gubaev, V. Zaverkin, P. Srinivasan, A. I. Duff, J. Kästner, and B. Grabowski, “Performance of two complementary machine-learned potentials in modelling chemically complex systems,” NPJ Computational Materials, vol. 9, p. 129, 2023, doi: 10.1038/s41524-023-01073-w.
- A. Straub, N. Karadimitriou, G. Reina, S. Frey, H. Steeb, and T. Ertl, “Visual Analysis of Displacement Processes in Porous Media using Spatio-Temporal Flow Graphs,” IEEE Transactions on Visualization and Computer Graphics, 2023, doi: 10.1109/TVCG.2023.3326931.
2022
- J. Potyka et al., “Towards DNS of Droplet-Jet Collisions of Immiscible Liquids with FS3D,” High Performance Computing in Science and Engineering ’22, Springer International Publishing, 2022. [Online]. Available: https://arxiv.org/abs/2212.09727
- M. Takamoto et al., “PDEBench: An Extensive Benchmark for Scientific Machine Learning,” in 36th Conference on Neural Information Processing Systems (NeurIPS 2022) Track on Datasets and Benchmarks, 2022.
- R. Leiteritz, P. Buchfink, B. Haasdonk, and D. Pflüger, “Surrogate-data-enriched Physics-Aware Neural Networks,” in Proceedings of the Northern Lights Deep Learning Workshop 2022, Mar. 2022. doi: 10.7557/18.6268.
- G. Tkachev, R. Cutura, M. Sedlmair, S. Frey, and T. Ertl, “Metaphorical Visualization: Mapping Data to Familiar Concepts,” in CHI Conference on Human Factors in Computing Systems Extended Abstracts, ACM, Apr. 2022. doi: 10.1145/3491101.3516393.
- T. Wenzel, M. Kurz, A. Beck, G. Santin, and B. Haasdonk, “Structured Deep Kernel Networks for Data-Driven Closure Terms of Turbulent Flows,” in Large-Scale Scientific Computing, I. Lirkov and S. Margenov, Eds., Cham: Springer International Publishing, 2022, pp. 410–418.
- B. Xiong, S. Zhu, N. Potyka, S. Pan, C. Zhou, and S. Staab, “Pseudo-Riemannian Graph Convolutional Networks,” in Advances in Neural Information Processing Systems, 2022. [Online]. Available: https://arxiv.org/abs/2106.03134
- A. Straub, S. Boblest, G. K. Karch, F. Sadlo, and T. Ertl, “Droplet-Local Line Integration for Multiphase Flow,” in 2022 IEEE Visualization and Visual Analytics (VIS), 2022, pp. 135–139. doi: 10.1109/VIS54862.2022.00036.
- J. Eirich, M. Münch, D. Jäckle, M. Sedlmair, J. Bonart, and T. Schreck, “RfX: A Design Study for the Interactive Exploration of a Random Forest to Enhance Testing Procedures for Electrical Engines,” Computer Graphics Forum, vol. 41, Art. no. 6, Mar. 2022, doi: 10.1111/cgf.14452.
- K. Pluhackova, V. Schittny, P.-C. Bürkner, C. Siligan, and A. Horner, “Multiple pore lining residues modulate water permeability of GlpF,” Protein Science, vol. 31, Art. no. 10, 2022, doi: https://doi.org/10.1002/pro.4431.
- V. Zaverkin, D. Holzmüller, I. Steinwart, and J. Kästner, “Exploring chemical and conformational spaces by batch mode deep active learning,” Digital Discovery, vol. 1, pp. 605–620, 2022, doi: 10.1039/D₂DD00034B.
- T. Munz, D. Väth, P. Kuznecov, N. T. Vu, and D. Weiskopf, “Visualization-based improvement of neural machine translation,” Computers & Graphics, vol. 103, pp. 45–60, 2022, doi: https://doi.org/10.1016/j.cag.2021.12.003.
- C. Arndt, A. Denker, J. Nickel, J. Leuschner, M. Schmidt, and G. Rigaud, “In Focus - hybrid deep learning approaches to the HDC2021 challenge,” Inverse Problems and Imaging, vol. 0, Art. no. 0, 2022, doi: 10.3934/ipi.2022061.
- S. Frey et al., “Visual Analysis of Two-Phase Flow Displacement Processes in Porous Media,” Computer graphics forum, vol. 41, Art. no. 1, 2022, doi: 10.1111/cgf.14432.
- D. Holzmüller and I. Steinwart, “Training two-layer ReLU networks with gradient descent is inconsistent,” Journal of Machine Learning Research, vol. 23, Art. no. 181, 2022, [Online]. Available: http://jmlr.org/papers/v23/20-830.html
- V. Zaverkin, D. Holzmüller, R. Schuldt, and J. Kästner, “Predicting properties of periodic systems from cluster data: A case study of liquid water,” The Journal of Chemical Physics, vol. 156, Art. no. 11, 2022, doi: 10.1063/5.0078983.
- V. Zaverkin, J. Netz, F. Zills, A. Köhn, and J. Kästner, “Thermally Averaged Magnetic Anisotropy Tensors via Machine Learning Based on Gaussian Moments,” Journal of Chemical Theory and Computation, vol. 18, pp. 1–12, 2022, doi: 10.1021/acs.jctc.1c00853.
2021
- T. Munz, D. Väth, P. Kuznecov, T. Vu, and D. Weiskopf, “Visual-Interactive Neural Machine Translation,” in Graphics Interface 2021, 2021. [Online]. Available: https://openreview.net/forum?id=DQHaCvN9xd
- R. Leiteritz, M. Hurler, and D. Pflüger, “Learning Free-Surface Flow with Physics-Informed Neural Networks,” in 2021 20th IEEE International Conference on Machine Learning and Applications (ICMLA), 2021, pp. 1664–1669. doi: 10.1109/ICMLA52953.2021.00266.
- D. Väth, P. Tilli, and N. T. Vu, “Beyond Accuracy: A Consolidated Tool for Visual Question Answering Benchmarking,” in Proceedings of the 2021 Conference on Empirical Methods in Natural Language Processing: System Demonstrations, 2021, pp. 114–123.
- D. Holzmüller and D. Pflüger, “Fast Sparse Grid Operations Using the Unidirectional Principle: A Generalized and Unified Framework,” in Sparse Grids and Applications - Munich 2018, H.-J. Bungartz, J. Garcke, and D. Pflüger, Eds., Cham: Springer International Publishing, 2021, pp. 69–100.
- D. Holzmüller, “On the Universality of the Double Descent Peak in Ridgeless Regression,” in International Conference on Learning Representations, 2021. [Online]. Available: https://openreview.net/forum?id=0IO5VdnSAaH
- A. Straub, G. K. Karch, F. Sadlo, and T. Ertl, “Implicit Visualization of 2D Vector Field Topology for Periodic Orbit Detection,” in Topological Methods in Data Analysis and Visualization VI, I. Hotz, T. Bin Masood, F. Sadlo, and J. Tierny, Eds., in Mathematics and Visualization. , Springer International Publishing, 2021, pp. 159–180. doi: 10.1007/978-3-030-83500-2_9.
- G. Tkachev, S. Frey, and T. Ertl, “S4: Self-Supervised learning of Spatiotemporal Similarity,” IEEE Transactions on Visualization and Computer Graphics, 2021.
- V. Zaverkin, D. Holzmüller, I. Steinwart, and J. Kästner, “Fast and Sample-Efficient Interatomic Neural Network Potentials for Molecules and Materials Based on Gaussian Moments,” Journal of Chemical Theory and Computation, vol. 17, Art. no. 10, 2021, doi: 10.1021/acs.jctc.1c00527.
- R. Garcia, T. Munz, and D. Weiskopf, “Visual analytics tool for the interpretation of hidden states in recurrent neural networks,” Visual Computing for Industry, Biomedicine, and Art, vol. 4, Art. no. 24, Sep. 2021, doi: 10.1186/s42492-021-00090-0.
- V. Zaverkin and J. Kästner, “Exploration of transferable and uniformly accurate neural network interatomic potentials using optimal experimental design,” Machine Learning: Science and Technology, vol. 2, Art. no. 3, 2021.
- K. Schatz et al., “2019 IEEE Scientific Visualization Contest Winner: Visual Analysis of Structure Formation in Cosmic Evolution,” IEEE Computer Graphics and Applications, vol. 41, Art. no. 6, 2021, doi: 10.1109/MCG.2020.3004613.
- M. Heinemann, S. Frey, G. Tkachev, A. Straub, F. Sadlo, and T. Ertl, “Visual analysis of droplet dynamics in large-scale multiphase spray simulations,” Journal of Visualization, vol. 24, Art. no. 5, May 2021, doi: 10.1007/s12650-021-00750-6.
2020
- E. Sood, S. Tannert, D. Frassinelli, A. Bulling, and N. T. Vu, “Interpreting Attention Models with Human Visual Attention in Machine Reading Comprehension,” in Proceedings of the 24th Conference on Computational Natural Language Learning, Online: Association for Computational Linguistics, Nov. 2020, pp. 12–25. doi: 10.18653/v1/2020.conll-1.2.
- T. Munz, N. Schaefer, T. Blascheck, K. Kurzhals, E. Zhang, and D. Weiskopf, “Demo of a Visual Gaze Analysis System for Virtual Board Games,” in ACM Symposium on Eye Tracking Research and Applications, in ETRA ’20 Adjunct. Stuttgart, Germany: Association for Computing Machinery, 2020. doi: 10.1145/3379157.3391985.
- F. Heyen et al., “ClaVis: An Interactive Visual Comparison System for Classifiers,” in Proceedings of the International Conference on Advanced Visual Interfaces, in AVI ’20. Salerno, Italy: Association for Computing Machinery, 2020. doi: 10.1145/3399715.3399814.
- G. Molpeceres, V. Zaverkin, and J. Kästner, “Neural-network assisted study of nitrogen atom dynamics on amorphous solid water – I. adsorption and desorption,” Mon. Not. R. Astron. Soc., vol. 499, pp. 1373–1384, 2020, doi: 10.1093/mnras/staa2891.
- V. Zaverkin and J. Kästner, “Gaussian Moments as Physically Inspired Molecular Descriptors for Accurate and Scalable Machine Learning Potentials,” Journal of Chemical Theory and Computation, vol. 16, pp. 5410–5421, 2020, doi: 10.1021/acs.jctc.0c00347.
- D. F. B. Häufle, I. Wochner, D. Holzmüller, D. Drieß, M. Günther, and S. Schmitt, “Muscles reduce neuronal information load : quantification of control effort in biological vs. robotic pointing and walking,” Frontiers in Robotics and AI, vol. 7, p. 77, 2020, doi: 10.3389/frobt.2020.00077.
- T. Munz, N. Schäfer, T. Blascheck, K. Kurzhals, E. Zhang, and D. Weiskopf, “Comparative Visual Gaze Analysis for Virtual Board Games,” The 13th International Symposium on Visual Information Communication and Interaction (VINCI 2020), 2020, doi: 10.1145/3430036.3430038.
2019
- T. Munz, M. Burch, T. van Benthem, Y. Poels, F. Beck, and D. Weiskopf, “Overlap-Free Drawing of Generalized Pythagoras Trees for Hierarchy Visualization,” in 2019 IEEE Visualization Conference (VIS), Oct. 2019, pp. 251–255. doi: 10.1109/VISUAL.2019.8933606.
- T. Munz, L. L. Chuang, S. Pannasch, and D. Weiskopf, “VisME: Visual microsaccades explorer,” Journal of Eye Movement Research, vol. 12, Art. no. 6, Dec. 2019, doi: 10.16910/jemr.12.6.5.
- G. Tkachev, S. Frey, and T. Ertl, “Local Prediction Models for Spatiotemporal Volume Visualization,” IEEE Transactions on Visualization and Computer Graphics, 2019, doi: 10.1109/TVCG.2019.2961893.
- I. Tabiai et al., “Hybrid image processing approach for autonomous crack area detection and tracking using local digital image correlation results applied to single-fiber interfacial debonding,” Engineering Fracture Mechanics, vol. 216, p. 106485, 2019, doi: 10.1016/j.engfracmech.2019.106485.
Software PN 6
2024
- T. Munz-Körner and D. Weiskopf, “Visual Analysis System to Explore the Visual Quality of Multidimensional Time Series Projections.” DaRUS, 2024. doi: 10.18419/DARUS-3553.
- T. Munz-Körner and D. Weiskopf, “Supplemental Material for “Exploring Visual Quality of Multidimensional Time Series Projections.”” DaRUS, 2024. doi: 10.18419/DARUS-3965.
2023
- D. Holzmüller, V. Zaverkin, J. Kästner, and I. Steinwart, “Code and Data for: A Framework and Benchmark for Deep Batch Active Learning for Regression arXiv v3.” 2023. doi: 10.18419/darus-3394.
- P. Rodegast, S. Maier, J. Kneifl, and J. C. Fehr, “Simulation Data from Motorcycle Sensors in Operational and Crash Scenarios.” 2023. doi: 10.18419/darus-3301.
- J. Kneifl and J. C. Fehr, “Crash Simulations of a Racing Kart’s Structural Frame Colliding against a Rigid Wall.” 2023. doi: 10.18419/darus-3789.
- N. Schäfer et al., “Visual Analysis System for Scene-Graph-Based Visual Question Answering.” 2023. doi: 10.18419/darus-3589.
2022
- D. Holzmüller, V. Zaverkin, J. Kästner, and I. Steinwart, “Code and Data for: A Framework and Benchmark for Deep Batch Active Learning for Regression arXiv v2.” 2022. doi: 10.18419/darus-3110.
- T. Munz, D. Väth, P. Kuznecov, N. T. Vu, and D. Weiskopf, “NMTVis - Extended Neural Machine Translation Visualization System.” 2022. doi: 10.18419/darus-2124.
- D. Holzmüller, “Replication Data for: Fast Sparse Grid Operations using the Unidirectional Principle: A Generalized and Unified Framework.” 2022. doi: 10.18419/darus-1779.
- G. Tkachev, “PyPlant: A Python Framework for Cached Function Pipelines.” 2022. doi: 10.18419/darus-2249.
- D. Holzmüller, V. Zaverkin, J. Kästner, and I. Steinwart, “Code and Data for: A Framework and Benchmark for Deep Batch Active Learning for Regression arXiv v1.” 2022. doi: 10.18419/darus-2615.
2021
- V. Zaverkin, D. Holzmüller, I. Steinwart, and J. Kästner, “Code for: Fast and Sample-Efficient Interatomic Neural Network Potentials for Molecules and Materials Based on Gaussian Moments.” 2021. doi: 10.18419/darus-2136.
- T. Munz, D. Väth, P. Kuznecov, N. T. Vu, and D. Weiskopf, “NMTVis - Neural Machine Translation Visualization System.” 2021. doi: 10.18419/darus-1849.
- G. Tkachev, “Replication Data for: “S4: Self-Supervised learning of Spatiotemporal Similarity.”” 2021. doi: 10.18419/darus-2174.
- T. Munz, R. Garcia, and D. Weiskopf, “Visual Analytics System for Hidden States in Recurrent Neural Networks.” DaRUS, 2021. doi: 10.18419/DARUS-2052.
2020
- J. Kneifl and J. Fehr, “Deformation of a Structural Frame of a Racing Kart Colliding against a Rigid Wall.” 2020. doi: 10.18419/darus-1150.
2019
- T. Munz, “VisME software v1.2.” Zenodo, 2019. doi: 10.5281/ZENODO.3352236.
Data PN 6
2024
- F. Bechler, “Simulation Results from the Descriptive Graph-based Model of two Example Driving Scenarios.” 2024. doi: 10.18419/darus-4116.
2023
- V. Zaverkin, D. Holzmüller, L. Bonfirraro, and J. Kästner, “Pre-trained and fine-tuned ANI models for: Transfer learning for chemically accurate interatomic neural network potentials.” 2023. doi: 10.18419/darus-3299.
- T. Munz-Körner, S. Künzel, and D. Weiskopf, “Supplemental Material for “Visual-Explainable AI: The Use Case of Language Models.”” 2023. doi: 10.18419/darus-3456.
- N. Schäfer et al., “Model Parameters and Evaluation Data for our Visual Analysis System for Scene-Graph-Based Visual Question Answering.” 2023. doi: 10.18419/darus-3597.
- M. Steffen, “Registered Cars and Motorized Two- and Three-wheelers in Worldwide Countries.” 2023. doi: 10.18419/darus-3378.
- M. Kelm, C. Bringedal, and B. Flemisch, “Replication Data for phase-field contributions in level-set comparison study.” 2023. doi: 10.18419/darus-3359.
2022
- M. Takamoto et al., “PDEBench Pretrained Models : Pretrained models for “PDEBench: An Extensive Benchmark for Scientific Machine Learning.”” 2022. doi: 10.18419/darus-2987.
- M. Takamoto et al., “PDEBench Datasets : Data for “PDEBench: An Extensive Benchmark for Scientific Machine Learning.”” 2022. doi: 10.18419/darus-2986.
- T. Praditia, M. Karlbauer, S. Otte, S. Oladyshkin, M. V. Butz, and W. Nowak, “Replication Data for: Learning Groundwater Contaminant Diffusion-Sorption Processes with a Finite Volume Neural Network.” 2022. doi: 10.18419/darus-3249.
2021
- S. Schulz, C. Bringedal, and S. Ackermann, “Code for relative permeabilities for two-phase flow between parallel plates with slip conditions.” 2021. doi: 10.18419/darus-2241.
- T. Munz, D. Väth, P. Kuznecov, N. T. Vu, and D. Weiskopf, “NMTVis - Trained Models for our Visual Analytics System.” DaRUS, 2021. doi: 10.18419/DARUS-1850.
2020
- T. Praditia, “Trained ANN Parameters for Physics-inspired Artificial Neural Network for Dynamic System.” 2020. doi: 10.18419/darus-634.
- T. Praditia, “Input-Output Dataset for Physics-inspired Artificial Neural Network for Dynamic System.” 2020. doi: 10.18419/darus-633.
- T. Munz, N. Schäfer, T. Blascheck, K. Kurzhals, E. Zhang, and D. Weiskopf, “Supplemental Material for Comparative Visual Gaze Analysis for Virtual Board Games.” DaRUS, 2020. doi: 10.18419/DARUS-1130.
Project Network Coordinators
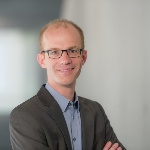
Dirk Pflüger
Prof. Dr. rer. nat.Scientific Computing | Vice-Head of GS SimTech
[Image: SimTech/Max Kovalenko]
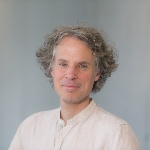
Ingo Steinwart
Univ.-Prof. Dr. rer. nat.Stochastics
[Image: SimTech/Max Kovalenko]
Mathias Niepert
Prof. Dr.Machine Learning for Simulation Science