Publications PN 5
2025
- A. Barth and A. Stein, “A stochastic transport problem with Lévy noise: Fully discrete numerical approximation.,” Mathematics and Computers in Simulation, vol. 227, pp. 347–370, 2025, [Online]. Available: https://doi.org/10.1016/j.matcom.2024.07.036
- E. Vahabzadeh, I. Buntic, F. Nazari, B. Flemisch, R. Helmig, and V. Niasar, “Applicability of the Vertical Equilibrium model to underground hydrogen injection and withdrawal,” International Journal of Hydrogen Energy, vol. 106, pp. 790–805, 2025, doi: https://doi.org/10.1016/j.ijhydene.2025.01.201.
- C. Riethmüller, E. Storvik, J. W. Both, and F. A. Radu, “Well-posedness analysis of the Cahn–Hilliard–Biot model,” Nonlinear Analysis: Real World Applications, vol. 84, p. 104271, Aug. 2025, doi: 10.1016/j.nonrwa.2024.104271.
2024 (submitted)
- W. Nowak, T. Brünnette, M. Schalkers, and M. Möller, “Overdispersion in gate tomography: Experiments and continuous, two-scale random walk model on the Bloch sphere,” ACM Transactions on Quantum Computing.
- F. Ejaz, N. Wildt, T. Wöhling, and W. Nowak, “Estimating total groundwater storage and its associated uncertainty through spatiotemporal Kriging of groundwater-level data,” Journal of Hydrology.
- R. Kohlhaas, J. Hommel, F. Weinhardt, H. Class, S. Oladyshkin, and B. Flemisch, “Numerical Investigation of Preferential Flow Paths in Enzymatically Induced Calcite Precipitation Supported by Bayesian Model Analysis,” Transport in Porous Media.
2024 (accepted)
- T. Xu, S. Xiao, S. Reuschen, N. Wildt, H.-J. H. Franssen, and W. Nowak, “Towards a community-wide effort for benchmarking in subsurface hydrological inversion: benchmarking cases, high-fidelity reference solutions, procedure and a first comparison,” Hydrology and Earth System Sciences, doi: 10.5194/hess-2024-60.
2024
- S. Xiao and W. Nowak, “Failure probability estimation with failure samples: An extension of the two-stage Markov chain Monte Carlo simulation,” Mechanical Systems and Signal Processing, vol. 212, p. 111300, Feb. 2024, doi: https://doi.org/10.1016/j.ymssp.2024.111300.
- A. Bøllingtoft, P. L. Bjerg, V. Rønde, N. Tuxen, W. Nowak, and M. Troldborg, “Quantification of contaminant mass discharge and uncertainties: Method and challenges in application at contaminated sites,” Journal of Contaminant Hydrology, vol. 268, p. 104453, Nov. 2024, doi: 10.1016/j.jconhyd.2024.104453.
- W.-P. Düll, B. Hilder, and G. Schneider, “Analysis of the embedded cell method in 2D for the numerical homogenization of metal-ceramic composite materials,” Journal of Applied Analysis, 2024, doi: doi:10.1515/jaa-2023-0124.
- C. Homs-Pons et al., “Coupled Simulation and Parameter Inversion for Neural System and Electrophysiological Muscle Models,” GAMM-Mitteilungen, Mar. 2024, doi: 10.1002/gamm.202370009.
- S. Gilg and G. Schneider, “Approximation of a two-dimensional Gross–Pitaevskii equation with a periodic potential in the tight-binding limit,” Mathematische Nachrichten, vol. n/a, Art. no. n/a, 2024, doi: https://doi.org/10.1002/mana.202300322.
- B. Hilder and U. Sharma, “Quantitative coarse-graining of Markov chains,” SIAM J. Math. Anal., vol. 56, Art. no. 1, 2024, doi: 10.1137/22M1473996.
- M. Oesting and O. Wintenberger, “Estimation of the spectral measure from convex combinations of regularly varying random vectors,” The Annals of Statistics, vol. 52, Art. no. 6, 2024, doi: 10.1214/24-AOS2387.
- M. Boon, I. Buntic, K. Ahmed, N. Dopffel, C. Peters, and H. Hajibeygi, “Microbial induced wettability alteration with implications for Underground Hydrogen Storage,” Scientific Reports, vol. 14, Art. no. 1, 2024, doi: 10.1038/s41598-024-58951-6.
- F. Huber, P.-C. Bürkner, D. Göddeke, and M. Schulte, “Knowledge-based modeling of simulation behavior for Bayesian optimization,” Computational Mechanics, vol. 74, Art. no. 1, Jul. 2024, doi: 10.1007/s00466-023-02427-3.
- B. Maier, D. Göddeke, F. Huber, T. Klotz, O. Röhrle, and M. Schulte, “OpenDiHu: An Efficient and Scalable Framework for Biophysical Simulations of the Neuromuscular System,” Journal of Computational Science, vol. 79, 2024, doi: https://doi.org/10.1016/j.jocs.2024.102291.
- I. Giannoulis, B. Schmidt, and G. Schneider, “NLS approximation for a scalar FPUT system on a 2D square lattice with a cubic nonlinearity,” J. Math. Anal. Appl., vol. 540, Art. no. 2, 2024, doi: 10.1016/j.jmaa.2024.128625.
- Y. Miao, C. Rohde, and H. Tang, “Well-posedness for a stochastic Camassa-Holm type equation with higher order nonlinearities,” Stochastic Partial Differential Equations: Analysis & Computation, vol. 12, Art. no. 1, 2024, doi: 10.1007/s40072-023-00291-z.
- J. Bartsch, P. Knopf, S. Scheurer, and J. Weber, “Controlling a Vlasov-Poisson Plasma by a Particle-in-Cell Method based on a Monte Carlo Framework,” SIAM Journal on Control and Optimization, vol. 62, Art. no. 4, Jul. 2024, doi: 10.1137/23M1563852.
2023
- D. Schneider, T. Schrader, and B. Uekermann, “Data-Parallel Radial-Basis Function Interpolation in preCICE,” in 10th edition of the International Conference on Computational Methods for Coupled Problems in Science and Engineering, M. Papadrakakis, S. B., and O. E., Eds., in COUPLED PROBLEMS 2023. CIMNE, 2023. doi: 10.23967/c.coupled.2023.016.
- J. Rettberg et al., “Port-Hamiltonian fluid–structure interaction modelling and structure-preserving model order reduction of a classical guitar,” Mathematical and Computer Modelling of Dynamical Systems, vol. 29, Art. no. 1, 2023, doi: 10.1080/13873954.2023.2173238.
- K. Mouris et al., “Stability criteria for Bayesian calibration of reservoir sedimentation models,” Modeling Earth Systems and Environment, 2023, doi: 10.1007/s40808-023-01712-7.
- G. Chourdakis, D. Schneider, and B. Uekermann, “OpenFOAM-preCICE: Coupling OpenFOAM with External Solvers for Multi-Physics Simulations,” OpenFOAM® Journal, vol. 3, pp. 1–25, Feb. 2023, doi: 10.51560/ofj.v3.88.
- P.-C. Bürkner, I. Kröker, S. Oladyshkin, and W. Nowak, “The sparse Polynomial Chaos expansion: a fully Bayesian approach with joint priors on the coefficients and global selection of terms,” Journal of Computational Physics, p. 112210, 2023, doi: https://doi.org/10.1016/j.jcp.2023.112210.
- S. Oladyshkin, T. Praditia, I. Kroeker, F. Mohammadi, W. Nowak, and S. Otte, “The Deep Arbitrary Polynomial Chaos Neural Network or how Deep Artificial Neural Networks could benefit from Data-Driven Homogeneous Chaos Theory,” Neural Networks, vol. 166, pp. 85–104, Sep. 2023, doi: 10.1016/j.neunet.2023.06.036.
- M. F. Morales Oreamuno, S. Oladyshkin, and W. Nowak, “Information-Theoretic Scores for Bayesian Model Selection and Similarity Analysis: Concept and Application to a Groundwater Problem,” Water Resources Research, vol. 59, Art. no. 7, Jul. 2023, doi: 10.1029/2022WR033711.
- L. Zhang, W. Nowak, S. Oladyshkin, Y. Wang, and J. Cai, “Opportunities and challenges in $CO_2$ geologic utilization and storage,” Advances in Geo-Energy Research, vol. 8, Art. no. 3, Jul. 2023, [Online]. Available: https://doi.org/10.46690/ager.2023.06.01
- R. Kohlhaas, I. Kröker, S. Oladyshkin, and W. Nowak, “Gaussian active learning on multi-resolution arbitrary polynomial chaos emulator: concept for bias correction, assessment of surrogate reliability and its application to the carbon dioxide benchmark,” Computational Geosciences, vol. 27, Art. no. 3, 2023, doi: doi:10.1007/s10596-023-10199-1.
- M. Oesting, A. Rapp, and E. Spodarev, “Detection of long range dependence in the time domain for (in)finite-variance time series,” Statistics, pp. 1–28, Dec. 2023, doi: 10.1080/02331888.2023.2287749.
- P. Buchfink, S. Glas, and B. Haasdonk, “Symplectic Model Reduction of Hamiltonian Systems on Nonlinear Manifolds and Approximation with Weakly Symplectic Autoencoder,” SIAM Journal on Scientific Computing, vol. 45, Art. no. 2, Mar. 2023, doi: 10.1137/21m1466657.
- F. Ejaz, A. Guthke, T. Wöhling, and W. Nowak, “Comprehensive uncertainty analysis for surface water and groundwater projections under climate change based on a lumped geo-hydrological model,” Journal of Hydrology, vol. 626, 2023, doi: https://doi.org/10.1016/j.jhydrol.2023.130323.
- I. Desai, E. Scheurer, C. Bringedal, and B. Uekermann, “Micro Manager: a Python package for adaptive and flexible two-scale coupling,” Journal of Open Source Software, vol. 8, Art. no. 91, Nov. 2023, doi: 10.21105/joss.05842.
- C. C. Horuz et al., “Physical Domain Reconstruction with Finite Volume Neural Networks,” Applied Artificial Intelligence, vol. 37, Art. no. 1, 2023, doi: https://doi.org/10.1080/08839514.2023.2204261.
- R. Fukuizumi, Y. Gao, G. Schneider, and M. Takahashi, “Pattern formation in 2D stochastic anisotropic Swift-Hohenberg equation,” Interdiscip. Inform. Sci., vol. 29, Art. no. 1, 2023, doi: 10.4036/iis.2023.a.03.
- R. Merkle and A. Barth, “On Properties and Applications of Gaussian Subordinated Lévy Fields,” Methodology and Computing in Applied Probability, vol. 25, p. 62, 2023, doi: 10.1007/s11009-023-10033-2.
- H. Sharma, H. Mu, P. Buchfink, R. Geelen, S. Glas, and B. Kramer, “Symplectic model reduction of Hamiltonian systems using data-driven quadratic manifolds,” Computer Methods in Applied Mechanics and Engineering, vol. 417, p. 116402, Dec. 2023, doi: 10.1016/j.cma.2023.116402.
- I. Kröker, S. Oladyshkin, and I. Rybak, “Global sensitivity analysis using multi-resolution polynomial chaos expansion for coupled Stokes-Darcy flow problems,” Computational Geosciences, 2023, doi: 10.1007/s10596-023-10236-z.
- S. Schwindt et al., “Bayesian calibration points to misconceptions in three-dimensional hydrodynamic reservoir modelling,” Water Resources Research, vol. 59, p. e2022WR033660, 2023, doi: https://doi.org/10.1029/2022WR033660.
2022
- S. Shuva, P. Buchfink, O. Röhrle, and B. Haasdonk, “Reduced Basis Methods for Efficient Simulation of a Rigid Robot Hand Interacting with Soft Tissue,” in Large-Scale Scientific Computing, I. Lirkov and S. Margenov, Eds., Springer International Publishing, 2022, pp. 402–409.
- M. Takamoto et al., “PDEBench: An Extensive Benchmark for Scientific Machine Learning,” in 36th Conference on Neural Information Processing Systems (NeurIPS 2022) Track on Datasets and Benchmarks, 2022.
- M. Karlbauer, T. Praditia, S. Otte, S. Oladyshkin, W. Nowak, and M. V. Butz, “Composing Partial Differential Equations with Physics-Aware Neural Networks,” in Proceedings of the 39th International Conference on Machine Learning, in Proceedings of Machine Learning Research. Baltimore, USA, 2022.
- P. Buchfink, S. Glas, and B. Haasdonk, “Optimal Bases for Symplectic Model Order Reduction of Canonizable Linear Hamiltonian Systems,” in IFAC-PapersOnLine, 2022, pp. 463–468. doi: 10.1016/j.ifacol.2022.09.138.
- C. C. Horuz et al., “Inferring Boundary Conditions in Finite Volume Neural Networks,” in International Conference on Artificial Neural Networks and Machine Learning -- ICANN 2022, E. Pimenidis, P. Angelov, C. Jayne, A. Papaleonidas, and M. Aydin, Eds., Cham: Springer International Publishing, 2022, pp. 538–549. doi: https://doi.org/10.1007/978-3-031-15919-0_45.
- R. Leiteritz, P. Buchfink, B. Haasdonk, and D. Pflüger, “Surrogate-data-enriched Physics-Aware Neural Networks,” in Proceedings of the Northern Lights Deep Learning Workshop 2022, Mar. 2022. doi: 10.7557/18.6268.
- S. Xiao and W. Nowak, “Reliability sensitivity analysis based on a two-stage Markov chain Monte Carlo simulation,” Aerospace Science and Technology, vol. 130, p. 107938, 2022, doi: 10.1016/j.ast.2022.107938.
- I. Banerjee, P. Walter, A. Guthke, K. G. Mumford, and W. Nowak, “The Method of Forced Probabilities: A Computation Trick for Bayesian Model Evidence,” Computational Geosciences, 2022, doi: 10.1007/s10596-022-10179-x.
- A. Schäfer Rodrigues Silva et al., “Diagnosing Similarities in Probabilistic Multi-Model Ensembles - an Application to Soil-Plant-Growth-Modeling,” Modeling Earth Systems and Environment, vol. 8, pp. 5143–5175, 2022, doi: 10.1007/s40808-022-01427-1.
- R. Merkle and A. Barth, “Subordinated Gaussian random fields in elliptic partial differential equations,” Stochastics and Partial Differential Equations: Analysis and Computations, vol. 11, pp. 819–867, 2022, doi: 10.1007/s40072-022-00246-w.
- A. Barth and A. Stein, “Numerical analysis for time-dependent advection-diffusion problems with random discontinuous coefficients,” ESAIM: M2AN, vol. 56, Art. no. 5, 2022, doi: 10.1051/m2an/2022054.
- G. Schneider and M. Winter, “The amplitude system for a simultaneous short-wave Turing and long-wave Hopf instability,” Discrete Contin. Dyn. Syst. Ser. S, vol. 15, Art. no. 9, 2022, doi: 10.3934/dcdss.2021119.
- B. Becker, B. Guo, I. Buntic, B. Flemisch, and R. Helmig, “An adaptive hybrid vertical equilibrium/full dimensional model for compositional multiphase flow,” Water Resources Research, p. e2021WR030990, 2022, doi: 10.1029/2021WR030990.
- J. Eller, T. Sauerborn, B. Becker, I. Buntic, J. Gross, and R. Helmig, “Modeling Subsurface Hydrogen Storage With Transport Properties From Entropy Scaling Using the PC‐SAFT Equation of State,” Water Resources Research, vol. 58, Art. no. 4, 2022, doi: 10.1029/2021wr030885.
- T. Praditia, M. Karlbauer, S. Otte, S. Oladyshkin, M. V. Butz, and W. Nowak, “Learning Groundwater Contaminant Diffusion-Sorption Processes with a Finite Volume Neural Network,” Water Resources Research, vol. 58, Art. no. 12, 2022, doi: 10.1029/2022WR033149.
- K. Cheng, L. Z, S. Xiao, S. Oladyshkin, and W. Nowak, “Mixed covariance function Kriging model for uncertainty quantification,” International Journal for Uncertainty Quantification, vol. 12, Art. no. 3, 2022, doi: 10.1615/Int.J.UncertaintyQuantification.2021035851.
- R. Merkle and A. Barth, “On Some Distributional Properties of Subordinated Gaussian Random Fields,” Methodology and Computing in Applied Probability, vol. 24, pp. 2661–2688, 2022, doi: 10.1007/s11009-022-09958-x.
- L. Chavez Rodriguez, A. González-Nicolás, B. Ingalls, W. Nowak, S. Xiao, and H. Pagel, “Optimal design of experiments to improve the characterization of atrazine degradation pathways in soil,” European Journal of Soil Science, vol. 73, Art. no. 1, 2022, doi: 10.1111/ejss.13211.
- A. Rodriguez-Pretelin, E. Morales-Casique, and W. Nowak, “Optimization-based clustering of random fields for computationally efficient and goal-oriented uncertainty quantification: concept and demonstration for delineation of wellhead protection areas in transient aquifers,” Advances in Water Resources, vol. 162, p. 104146, 2022, doi: 10.1016/j.advwatres.2022.104146.
- V. Wagner, B. Castellaz, M. Oesting, and N. Radde, “Quasi-Entropy Closure: A Fast and Reliable Approach to Close the Moment Equations of the Chemical Master Equation,” Bioinformatics, vol. 38, Art. no. 18, 2022, doi: 10.1093/bioinformatics/btac501.
- R. Merkle and A. Barth, “Multilevel Monte Carlo estimators for elliptic PDEs with Lévy-type diffusion coefficient,” BIT Numerical Mathematics, vol. 62, pp. 1279–1317, 2022, doi: 10.1007/s10543-022-00912-4.
- A. Gonzalez-Nicolas et al., “Optimal Exposure Time in Gamma-Ray Attenuation Experiments for Monitoring Time-Dependent Densities,” Transport in Porous Media, vol. 143, Art. no. 2, 2022, doi: 10.1007/s11242-022-01777-5.
- I. Kröker and S. Oladyshkin, “Arbitrary Multi-Resolution Multi-Wavelet-based Polynomial Chaos Expansion for Data-Driven Uncertainty Quantification,” Reliability Engineering & System Safety, vol. 222, p. 108376, 2022, doi: 10.1016/j.ress.2022.108376.
- B. Maier and M. Schulte, “Mesh generation and multi-scale simulation of a contracting muscle–tendon complex,” Journal of Computational Science, vol. 59, p. 101559, 2022, doi: https://doi.org/10.1016/j.jocs.2022.101559.
- H.-f. Hsueh, A. Guthke, T. Wöhling, and W. Nowak, “Diagnosis of model-structural errors with a sliding time-window Bayesian analysis,” Water Resources Research, vol. 58, p. e2021WR030590, 2022, doi: doi:10.1029/2021WR030590.
- M. Oesting and K. Strokorb, “A Comparative Tour through the Simulation Algorithms for Max-Stable Processes,” Statistical Science, vol. 37, Art. no. 1, 2022, doi: 10.1214/20-STS820.
- M. Kurz, P. Offenhäuser, D. Viola, O. Shcherbakov, M. Resch, and A. Beck, “Deep reinforcement learning for computational fluid dynamics on HPC systems,” Journal of Computational Science, vol. 65, p. 101884, Nov. 2022, doi: 10.1016/j.jocs.2022.101884.
2021
- T. Praditia, M. Karlbauer, S. Otte, S. Oladyshkin, M. Butz, and W. Nowak, “Finite Volume Neural Network: Modeling Subsurface Contaminant Transport,” in Deep Learning for Simulation ICLR Workshop 2021, 2021. [Online]. Available: https://simdl.github.io/files/33.pdf
- J. Schmalfuss, C. Riethmüller, M. Altenbernd, K. Weishaupt, and D. Göddeke, “Partitioned coupling vs. monolithic block-preconditioning approaches for solving Stokes-Darcy systems,” in Proceedings of the International Conference on Computational Methods for Coupled Problems in Science and Engineering (COUPLED PROBLEMS), 2021. doi: 10.23967/coupled.2021.043.
- B. Maier, D. Schneider, M. Schulte, and B. Uekermann, “Bridging Scales with Volume Coupling --- Scalable Simulations of Muscle Contraction and Electromyography,” in High Performance Computing in Science and Engineering ’21: Transactions of the High Performance Computing Center, Stuttgart (HLRS) 2021, 2021.
- B. Rodenberg, I. Desai, R. Hertrich, A. Jaust, and B. Uekermann, “FEniCS–preCICE: Coupling FEniCS to other simulation software,” SoftwareX, vol. 16, p. 100807, Dec. 2021, doi: 10.1016/j.softx.2021.100807.
- M. Osorno, M. Schirwon, N. Kijanski, R. Sivanesapillai, H. Steeb, and D. Göddeke, “A cross-platform, high-performance SPH toolkit for image-based flow simulations on the pore scale of porous media,” Computer Physics Communications, vol. 267, Art. no. 108059, Oct. 2021, doi: 10.1016/j.cpc.2021.108059.
- S. Reuschen, A. Guthke, and W. Nowak, “The Four Ways to Consider Measurement Noise in Bayesian Model Selection - And Which One to Choose,” Water Resources Research, vol. 57, Art. no. 11, 2021.
- A. Krämer et al., “Multi-physics multi-scale HPC simulations of skeletal muscles,” High Performance Computing in Science and Engineering ’20: Transactions of the High Performance Computing Center, Stuttgart(HLRS) 2020, pp. 185–203, 2021, doi: 10.1007/978-3-030-80602-6_13.
- I. Banerjee, A. Guthke, C. J. C. Van de Ven, K. G. Mumford, and W. Nowak, “Overcoming the model-data-fit problem in porous media: A quantitative method to compare invasion-percolation models to high-resolution data,” Water Resources Research, vol. 57, Art. no. 7, 2021, doi: 10.1029/2021WR029986.
- S. Xiao, T. Praditia, S. Oladyshkin, and W. Nowak, “Global sensitivity analysis of a CaO/Ca(OH)2 thermochemical energy storage model for parametric effect analysis,” Applied Energy, vol. 285, p. 116456, 2021.
- S. Xiao, T. Xu, S. Reuschen, W. Nowak, and H.-J. H. Franssen, “Bayesian inversion of multi-Gaussian log-conductivity fields with uncertain hyperparameters: an extension of preconditioned Crank-Nicolson Markov chain Monte Carlo with parallel tempering,” Water Resources Research, vol. 57, p. e2021WR030313, 2021, doi: 10.1029/2021WR030313.
- S. Scheurer et al., “Surrogate-based Bayesian Comparison of Computationally Expensive Models: Application to Microbially Induced Calcite Precipitation,” Computational Geosciences, vol. 25, pp. 1899–1917, 2021.
- A. Rörich, T. A. Werthmann, D. Göddeke, and L. Grasedyck, “Bayesian inversion for electromyography using low-rank tensor formats,” Inverse Problems, vol. 37, Art. no. 5, Mar. 2021, doi: 10.1088/1361-6420/abd85a.
- K. Cheng, S. Xiao, X. Zhang, S. Oladyshkin, and W. Nowak, “Resampling method for reliability-based design optimization based on thermodynamic integration and parallel tempering,” Mechanical Systems and Signal Processing, vol. 156, p. 107630, 2021, doi: 10.1016/j.ymssp.2021.107630.
2020
- S. Oladyshkin et al., “Uncertainty quantification using Bayesian arbitrary polynomial chaos for computationally demanding environmental modelling: conventional, sparse and adaptive strategy,” in Computational Methods in Water Resources (CMWR), 2020.
- P. Buchfink, B. Haasdonk, and S. Rave, “PSD-Greedy Basis Generation for Structure-Preserving Model Order Reduction of Hamiltonian Systems,” in Proceedings of the Conference Algoritmy 2020, P. Frolkovič, K. Mikula, and D. Ševčovič, Eds., Vydavateľstvo SPEKTRUM, Aug. 2020, pp. 151–160. [Online]. Available: http://www.iam.fmph.uniba.sk/amuc/ojs/index.php/algoritmy/article/view/1577/829
- L. Brencher and A. Barth, “Hyperbolic Conservation Laws with Stochastic Discontinuous Flux Functions,” Finite Volumes for Complex Applications IX : Methods, Theoretical Aspects, Examples, Art. no. 323, 2020, doi: 10.1007/978-3-030-43651-3_23.
- D. Erdal, S. Xiao, W. Nowak, and O. Cirpka, “Sampling Behavioral Model Parameters for Ensemble-based Sensitivity Analysis using Gaussian Process Emulation and Active Subspaces,” Stochastic Environmental Research and Risk Assessment, vol. 34, pp. 1813–1830, 2020, doi: 10.1007/s00477-020-01867-0.
- I. Guisandez, J. I. Perez-Diaz, W. Nowak, and J. Haas, “Should environmental constraints be considered in linear programming based water value calculators?,” International Journal of Electrical Power & Energy Systems, vol. 117, Art. no. 105662, May 2020, doi: 10.1016/j.ijepes.2019.105662.
- M. Sinsbeck, M. Höge, and W. Nowak, “Exploratory-phase-free estimation of GP hyperparameters in sequential design methods - at the example of Bayesian inverse problems,” Frontiers in Artificial Intelligence, section AI in Food, Agriculture and Water, vol. 3, Art. no. 52, 2020, doi: 10.3389/frai.2020.00052.
- S. Xiao, S. Oladyshkin, and W. Nowak, “Reliability analysis with stratified importance sampling based on adaptive Kriging,” Reliability Engineering & System Safety, vol. 197, p. 106852, May 2020, doi: 10.1016/j.ress.2020.106852.
- T. Xu, S. Reuschen, W. Nowak, and H.-J. H. Franssen, “Preconditioned Crank-Nicolson Markov chain Monte Carlo coupled with parallel tempering: An efficient method for Bayesian inversion of multi-Gaussian log-hydraulic conductivity fields,” Water Resources Research, vol. 56, Art. no. 8, 2020, doi: 10.1029/2020WR027110.
- F. Beckers, A. Heredia, M. Noack, W. Nowak, S. Wieprecht, and S. Oladyshkin, “Bayesian Calibration and Validation of a Large-scale and Time-demanding Sediment Transport Model,” Water Resources Research, vol. 56, Art. no. 7, 2020, doi: 10.1029/2019WR026966.
- A. Schäfer Rodrigues Silva, A. Guthke, M. Höge, O. A. Cirpka, and W. Nowak, “Strategies for simplifying reactive transport models - a Bayesian model comparison,” Water Resources Research, vol. 56, p. e2020WR028100, 2020, doi: 10.1029/2020WR028100.
- S. Xiao, S. Oladyshkin, and W. Nowak, “Forward-reverse switch between density-based and regional sensitivity analysis,” Applied Mathematical Modelling, vol. 84, pp. 377–392, 2020.
2019
- S. Oladyshkin and W. Nowak, “The Connection between Bayesian Inference and Information Theory for Model Selection, Information Gain and Experimental Design,” Entropy, vol. 21, Art. no. 11, Nov. 2019, doi: 10.3390/e21111081.
- S. Xiao, S. Reuschen, G. Köse, S. Oladyshkin, and W. Nowak, “Estimation of small failure probabilities based on thermodynamic integration and parallel tempering,” Mechanical Systems and Signal Processing, vol. 133, p. 106248, Nov. 2019, doi: 10.1016/j.ymssp.2019.106248.
Software PN 5
2023
- R. Kohlhaas, I. Kröker, S. Oladyshkin, and W. Nowak, “Gaussian active learning on multi-resolution arbitrary polynomial chaos emulator : Data and Software.” 2023. doi: 10.18419/darus-2829.
Data PN 5
2023
- J. Rettberg et al., “Replication Data for: Port-Hamiltonian Fluid-Structure Interaction Modeling and Structure-Preserving Model Order Reduction of a Classical Guitar.” 2023. doi: 10.18419/darus-3248.
Project Network Coordinators
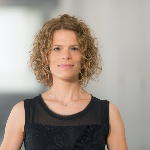
Andrea Barth
Prof. Dr.Computational Methods for Uncertainty Quantification
[Image: SimTech/Max Kovalenko]
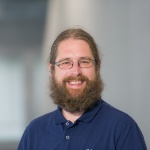
Dominik Göddeke
Prof. Dr. rer. nat.Computational Mathematics for Complex Simulations in Science and Engineering
[Image: SimTech/Max Kovalenko]