Particle and continuum methods are established tools for the simulation of materials, biological matter, and technical processes. However, isolated approaches in these fields face substantial problems, e.g., for predictive materials design from first principles. Thus, the overarching goal of this Project Network is to develop novel methods for data integration into particle and continuum simulation models. Specifically, the predictive power of model, dimensionality, or complexity reduction in materials science and biotechnology will be enhanced by incorporating data from different sources, including experiments, simulations, or rapidly growing available databases. In contrast to standard parameter identification across scales, PN 3 will pioneer a holistic integration of data into model reduction techniques and machine-learned models respecting physical constraints. Target applications beckon in several fields of engineering and sciences, and our methods will therefore contribute to each of our three Visionary Examples.
Research questions
RQ 1 Scale bridging for particle models: How can we realize scale-bridging and coarse-graining of particle models by data-assisted model reduction?
RQ 2 Complexity reduction in continuum models: How can we reduce complexity in continuumbased models by data-assisted model reduction?
RQ 3 Machine-learned models: How can we develop particle and continuum models based on machine learning from experimental and simulation data?
Ongoing projects
Completed projects
Ongoing associated projects
Completed associated projects
Project Network Coordinators
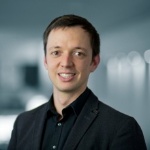
Felix Fritzen
Prof. Dr.-Ing. Dipl.-Math. techn.Data Analytics in Engineering
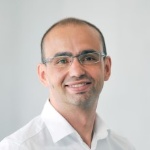
Blazej Grabowski
Prof. Dr. rer. nat.Materials Design
[Photo: (c) SimTech/Max Kovalenko]