Publications
2024
- S. Weirich, D. Kusevic, P. Schnee, J. Reiter, J. Pleiss, and A. Jeltsch, “Discovery of NSD2 non-histone substrates and design of a super-substrate,” Communications Biology, vol. 7, no. 1, Art. no. 1, 2024, doi: 10.1038/s42003-024-06395-z.
- T. Siebert, Ed., “Determination of muscle shape deformations of the tibialis anterior during dynamic contractions using 3D ultrasound. Front. Bioeng. Biotechnol. 12:1388907.,” Frontiers in Bioengineering and Biotechnology, vol. 12, Jun. 2024, doi: 10.3389/fbioe.2024.1388907.
- A. S. Sahrmann, L. Vosse, T. Siebert, G. G. Handsfield, and O. Röhrle, “3D ultrasound-based determination of skeletal muscle fascicle orientations,” Biomechanics and Modeling in Mechanobiology, Mar. 2024, doi: 10.1007/s10237-024-01837-3.
- F. Neubauer, P. Bredl, M. Xu, K. Patel, J. Pleiss, and B. Uekermann, “MetaConfigurator: A User-Friendly Tool for Editing Structured Data Files,” Datenbank-Spektrum, vol. 24, pp. 161–169, 2024, doi: 10.1007/s13222-024-00472-7.
- S. M. Seyedpour, M. Azhdari, L. Lambers, T. Ricken, and G. Rezazadeh, “One-dimensional thermomechanical bio-heating analysis of viscoelastic tissue to laser radiation shapes,” International Journal of Heat and Mass Transfer, vol. 218, p. 124747, 2024, doi: https://doi.org/10.1016/j.ijheatmasstransfer.2023.124747.
- J. Pleiss, “FAIR Data and Software: Improving Efficiency and Quality of Biocatalytic Science,” ACS Catal., vol. 14, no. 4, Art. no. 4, Feb. 2024, doi: 10.1021/acscatal.3c06337.
- B. Flemisch et al., “Research Data Management in Simulation Science: Infrastructure, Tools, and Applications,” Datenbank-Spektrum, 2024, doi: https://doi.org/10.1007/s13222-024-00475-4.
- P. Schnee, J. Pleiss, and A. Jeltsch, “Approaching the catalytic mechanism of protein lysine methyltransferases by biochemical and simulation techniques,” Critical Reviews in Biochemistry and Molecular Biology, vol. 59, no. 1–2, Art. no. 1–2, 2024, doi: 10.1080/10409238.2024.2318547.
2023
- S. M. Seyedpour, L. Lambers, G. Rezazadeh, and T. Ricken, “Mathematical modelling of the dynamic response of an implantable enhanced capacitive glaucoma pressure sensor,” Measurement: Sensors, p. 100936, 2023, doi: https://doi.org/10.1016/j.measen.2023.100936.
- C. Guttà, C. Morhard, and M. Rehm, “Applying a GAN-based classifier to improve transcriptome-based prognostication in breast cancer,” PLOS Computational Biology, vol. 19, no. 4, Art. no. 4, Apr. 2023, doi: 10.1371/journal.pcbi.1011035.
- S. Lauterbach et al., “EnzymeML: seamless data flow and modeling of enzymatic data,” Nature Methods, vol. 20, no. 3, Art. no. 3, 2023, doi: 10.1038/s41592-022-01763-1.
- R. Christian, T. Andre, B. R., and S. Tobias, “Structurally motivated models to explain the muscle’s force-length relationship,” Biophysical Journal 1, vol. 122, no. 17, Art. no. 17, Sep. 2023, doi: 10.1016/j.bpj.2023.05.026.
- T. Walter, N. Stutzig, and T. Siebert, “Active exoskeleton reduces erector spinae muscle activity during lifting,” Frontiers in Bioengineering and Biotechnology, vol. 11, Apr. 2023, doi: 10.3389/fbioe.2023.1150170.
- M. Azhdari et al., “Non-local three phase lag bio thermal modeling of skin tissue and experimental evaluation,” International Communications in Heat and Mass Transfer, vol. 149, p. 107146, 2023, doi: https://doi.org/10.1016/j.icheatmasstransfer.2023.107146.
- L. Mandl, A. Mielke, S. M. Seyedpour, and T. Ricken, “Affine transformations accelerate the training of physics-informed neural networks of a one-dimensional consolidation problem,” Scientific Reports, vol. 13, no. 1, Art. no. 1, 2023, doi: 10.1038/s41598-023-42141-x.
- V. Wagner and N. Radde, “The impossible challenge of estimating non-existent moments of the Chemical Master Equation,” Bioinformatics, vol. 39, no. Supplement_1, Art. no. Supplement_1, Jun. 2023, doi: 10.1093/bioinformatics/btad205.
- T. Giess, S. Itzigehl, J. Range, R. Schömig, J. R. Bruckner, and J. Pleiss, “FAIR and scalable management of small-angle X-ray scattering data,” Journal of Applied Crystallography, vol. 56, no. 2, Art. no. 2, Apr. 2023, doi: 10.1107/S1600576723001577.
- K. Gubaev, V. Zaverkin, P. Srinivasan, A. I. Duff, J. Kästner, and B. Grabowski, “Performance of two complementary machine-learned potentials in modelling chemically complex systems,” NPJ Computational Materials, vol. 9, p. 129, 2023, doi: 10.1038/s41524-023-01073-w.
- L. Pfitzer, J. Heitkämper, J. Kästner, and R. Peters, “Use of the N–O Bonds in N-Mesyloxyamides and N-Mesyloxyimides To Gain Access to 5-Alkoxy-3,4-dialkyloxazol-2-ones and 3-Hetero-Substituted Succinimides: A Combined Experimental and Theoretical Study,” Synthesis, vol. 55, no. 26, Art. no. 26, 2023, doi: 10.1055/s-0042-1751447.
- V. Wagner, R. Strässer, F. Allgöwer, and N. E. Radde, “A provably convergent control closure scheme for the Method of Moments of the Chemical Master Equation,” Journal of Chemical Theory and Computation, vol. 19, no. 24, Art. no. 24, Dec. 2023, doi: https://doi.org/10.1021/acs.jctc.3c00548.
- S. Höpfl, J. Pleiss, and N. E. Radde, “Bayesian estimation reveals that reproducible models in Systems Biology get more citations,” Scientific reports, vol. 13, p. 2695, 2023, doi: 10.1038/s41598-023-29340-2.
2022
- C. Guttà, C. Morhard, and M. Rehm, “T-GAN-D: a GAN-based classifier for breast cancer prognostication.” Zenodo, Oct. 2022. doi: 10.5281/zenodo.7151831.
- F. Bertrand, M. Brodbeck, and T. Ricken, “On robust discretization methods for poroelastic problems: Numerical examples and counter-examples,” Examples and Counterexamples, vol. 2, p. 100087, Nov. 2022, doi: 10.1016/j.exco.2022.100087.
- C. Hagenlocher, R. Siebert, B. Taschke, S. Wieske, A. Hausser, and M. Rehm, “ER stress-induced cell death proceeds independently of the TRAIL-R2 signaling axis in pancreatic β cells,” Cell Death Discovery, vol. 8, no. 1, Art. no. 1, Jan. 2022, doi: 10.1038/s41420-022-00830-y.
- S. Weidner, A. Tomalka, C. Rode, and T. Siebert, “How velocity impacts eccentric force generation of fully activated skinned skeletal muscle fibers in long stretches,” Journal of Applied Physiology, vol. 133, no. 1, Art. no. 1, 2022, doi: 10.1152/japplphysiol.00735.2021.
- A. H. Ludwig-Słomczyńska and M. Rehm, “Mitochondrial genome variations, mitochondrial-nuclear compatibility, and their association with metabolic diseases,” Obesity, May 2022, doi: 10.1002/OBY.23424.
- J. Vera et al., “Melanoma 2.0. Skin cancer as a paradigm for emerging diagnostic technologies, computational modelling and artificial intelligence,” Briefings in Bioinformatics, Oct. 2022, doi: 10.1093/BIB/BBAC433.
- C. Boccellato and M. Rehm, “Glioblastoma, from disease understanding towards optimal cell-based in vitro models,” Cellular Oncology, Jun. 2022, doi: 10.1007/s13402-022-00684-7.
- V. Wagner, S. Höpfl, V. Klingel, M. C. Pop, and N. E. Radde, “An inverse transformation algorithm to infer parameter distributions from population snapshot data,” IFAC-PapersOnLine, vol. 55, no. 23, Art. no. 23, 2022, doi: https://doi.org/10.1016/j.ifacol.2023.01.020.
- A. Tomalka, M. Heim, A. Klotz, C. Rode, and T. Siebert, “Ultrastructural and kinetic evidence support that thick filaments slide through the Z-disc,” Interface : journal of the Royal Society, vol. 19, no. 197, Art. no. 197, 2022, doi: 10.1098/rsif.2022.0642.
- A. Mack et al., “Preferential Self-interaction of DNA Methyltransferase DNMT3A Subunits Containing the R882H Cancer Mutation Leads to Dominant Changes of Flanking Sequence Preferences,” Journal of Molecular Biology, vol. 434, no. 7, Art. no. 7, 2022, doi: 10.1016/j.jmb.2022.167482.
- V. Wagner, B. Castellaz, M. Oesting, and N. Radde, “Quasi-Entropy Closure: A Fast and Reliable Approach to Close the Moment Equations of the Chemical Master Equation,” Bioinformatics, vol. 38, no. 18, Art. no. 18, 2022, doi: 10.1093/bioinformatics/btac501.
- P. Schnee et al., “Mechanistic basis of the increased methylation activity of the SETD2 protein lysine methyltransferase towards a designed super-substrate peptide,” Communications Chemistry, vol. 5, no. 1, Art. no. 1, 2022, doi: 10.1038/s42004-022-00753-w.
- F. Kempter, L. Lantella, N. Stutzig, J. Fehr, and T. Siebert, “Role of Rotated Head Postures on Volunteer Kinematics and Muscle Activity in Braking Scenarios Performed on a Driving Simulator,” Annals of Biomedical Engineering, vol. 51, no. 4, Art. no. 4, 2022, doi: 10.1007/s10439-022-03087-9.
- J. Range et al., “EnzymeML—a data exchange format for biocatalysis and enzymology,” The FEBS Journal, vol. 289, no. 19, Art. no. 19, Oct. 2022, doi: https://doi.org/10.1111/febs.16318.
2021
- A. Armiti-Juber and T. Ricken, “Model order reduction for deformable porous materials in thin domains via asymptotic analysis,” Archive of Applied Mechanics, 2021, doi: 10.1007/s00419-021-01907-3.
- N. Krishna Moorthy et al., “Low-Level Endothelial TRAIL-Receptor Expression Obstructs the CNS-Delivery of Angiopep-2 Functionalised TRAIL-Receptor Agonists for the Treatment of Glioblastoma,” Molecules, vol. 26, no. 24, Art. no. 24, Dec. 2021, doi: 10.3390/MOLECULES26247582.
- S. M. Seyedpour et al., “Application of Magnetic Resonance Imaging in Liver Biomechanics: A Systematic Review,” Frontiers in Physiology, vol. 12, Sep. 2021, doi: 10.3389/fphys.2021.733393.
- C. Boccellato et al., “Marizomib sensitizes primary glioma cells to apoptosis induced by a latest-generation TRAIL receptor agonist,” Cell Death & Disease, vol. 12, no. 7, Art. no. 7, Jul. 2021, doi: 10.1038/s41419-021-03927-x.
- B. Christ et al., “Hepatectomy-Induced Alterations in Hepatic Perfusion and Function - Toward Multi-Scale Computational Modeling for a Better Prediction of Post-hepatectomy Liver Function,” Frontiers in Physiology, vol. 12, Nov. 2021, doi: 10.3389/fphys.2021.733868.
- W. Ehlers, M. Morrison (Rehm), P. Schröder, D. Stöhr, and A. Wagner, “Multiphasic modelling and computation of metastatic lung-cancer cell proliferation and atrophy in brain tissue based on experimental data,” Biomechanics and Modeling in Mechanobiology, 2021, doi: 10.1007/s10237-021-01535-4.
- J. Pleiss, “Standardized data, scalable documentation, sustainable storage – EnzymeML as a basis for FAIR data management in biocatalysis,” ChemCatChem, vol. 13, pp. 3909–3913, 2021, doi: https://doi.org/10.1002/cctc.202100822.
- A. Tomalka, S. Weidner, D. Hahn, W. Seiberl, and T. Siebert, “Power Amplification Increases With Contraction Velocity During Stretch-Shortening Cycles of Skinned Muscle Fibers,” Frontiers in Physiology, vol. 12, Mar. 2021, doi: 10.3389/fphys.2021.644981.
2020
- K. Kuritz, D. Stöhr, D. S. Maichl, N. Pollak, M. Rehm, and F. Allgöwer, “Reconstructing temporal and spatial dynamics from single-cell pseudotime using prior knowledge of real scale cell densities,” Scientific Reports, vol. 10, no. 1, Art. no. 1, Dec. 2020, doi: 10.1038/s41598-020-60400-z.
- D. Imig, N. Pollak, F. Allgöwer, and M. Rehm, “Sample-based modeling reveals bidirectional interplay between cell cycle progression and extrinsic apoptosis,” PLOS Computational Biology, vol. 16, no. 6, Art. no. 6, Jun. 2020, doi: 10.1371/journal.pcbi.1007812.
- S. Adam et al., “DNA sequence-dependent activity and base flipping mechanisms of DNMT1 regulate genome-wide DNA methylation,” Nature Communications, vol. 11, no. 1, Art. no. 1, 2020, doi: 10.1038/s41467-020-17531-8.
- D. Stöhr et al., “Stress-induced TRAILR2 expression overcomes TRAIL resistance in cancer cell spheroids,” Cell Death & Differentiation, no. 27, Art. no. 27, 2020, doi: 10.1038/s41418-020-0559-3.
- C. Guttà et al., “Low expression of pro-apoptotic proteins Bax, Bak and Smac indicates prolonged progression-free survival in chemotherapy-treated metastatic melanoma,” Cell Death & Disease, vol. 11, no. 2, Art. no. 2, Feb. 2020, doi: 10.1038/s41419-020-2309-3.
- D. Stöhr, A. Jeltsch, and M. Rehm, “TRAIL receptor signaling: From the basics of canonical signal transduction toward its entanglement with ER stress and the unfolded protein response.,” Cell Death Regulation in Health and Disease-Part A, p. 57, 2020.
- G. Fullstone, T. L. Bauer, C. Guttà, M. Salvucci, J. H. M. Prehn, and M. Rehm, “The apoptosome molecular timer synergises with XIAP to suppress apoptosis execution and contributes to prognosticating survival in colorectal cancer,” Cell Death & Differentiation, no. 27, Art. no. 27, 2020, doi: 10.1038/s41418-020-0545-9.
- A. Tomalka, S. Weidner, D. Hahn, W. Seiberl, and T. Siebert, “Cross-Bridges and Sarcomeric Non-cross-bridge Structures Contribute to Increased Work in Stretch-Shortening Cycles,” Frontiers in Physiology, vol. 11, Jul. 2020, doi: 10.3389/fphys.2020.00921.
- V. Vetma et al., “Convergence of pathway analysis and pattern recognition predicts sensitization to latest generation TRAIL therapeutics by IAP antagonism,” Cell Death & Differentiation, vol. 27, no. 8, Art. no. 8, Feb. 2020, doi: 10.1038/s41418-020-0512-5.
- D. Stöhr and M. Rehm, “Linking hyperosmotic stress and apoptotic sensitivity,” The FEBS Journal, p. febs.15520, Aug. 2020, doi: 10.1111/febs.15520.
- G. Fullstone, C. Guttà, A. Beyer, and M. Rehm, “The FLAME-accelerated signalling tool (FaST) for facile parallelisation of flexible agent-based models of cell signalling,” npj Systems Biology and Applications, vol. 6, no. 1, Art. no. 1, 2020, doi: 10.1038/s41540-020-0128-x.
2019
- A. Tomalka, O. Röhrle, J. C. Han, T. Pham, A. J. Taberner, and T. Siebert, “Extensive eccentric contractions in intact cardiac trabeculae: revealing compelling differences in contractile behaviour compared to skeletal muscles,” Proceedings of the Royal Society B, vol. 286, no. 1903, Art. no. 1903, 2019, doi: 10.1098/rspb.2019.0719.
Published software
2023
- P. F. Santana Chacon, M. Hammer, I. Wochner, J. R. Walter, and S. Schmitt, “Replication Data for: A physiologically enhanced muscle spindle model: using a Hill-type model for extrafusal fibers as template for intrafusal fibers.” 2023. doi: 10.18419/darus-3796.
- F. Kempter, L. Lantella, N. Stutzig, J. C. Fehr, and T. Siebert, “Neck Reflex Behavior in Driving Simulator Experiments - Academic-Scale Simulator at ITM.” 2023. doi: 10.18419/darus-3000.
2022
- J. Kneifl, J. Hay, and J. Fehr, “Human Occupant Motion in Pre-Crash Scenario.” 2022. doi: 10.18419/darus-2471.
- S. Schmitt, “demoa-base: a biophysics simulator for muscle-driven motion.” 2022. doi: 10.18419/darus-2550.
- I. Wochner and S. Schmitt, “MPC/OC Code for: Learning with Muscles: Benefits for Data-Efficiency and Robustness in Anthropomorphic Tasks.” 2022. doi: 10.18419/darus-3268.
Published data
2022
- M. Hammer, J. M. Riede, L. Meszaros-Beller, and S. Schmitt, “gspine: A Human Spine Model Built Using Literature Data : a human spine model built using literature data.” 2022. doi: 10.18419/darus-2814.
Project Network Coordinators
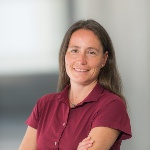
Nicole Radde
Prof. Dr. rer. nat.Mathematical Modeling and Simulation of Cellular Systems
[Photo: SimTech/Max Kovalenko]
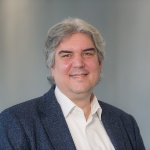
Oliver Röhrle
Prof.Continuum Biomechanics and Mechanobiology
[Photo: SimTech/Max Kovalenko]