We aim to achieve large-scale simulations of unprecedented reliability and efficiency in the triangle spanned by the accuracy demand for small systematic errors, the precision challenge to attain low stochastic errors, and the resource limitations imposed by computing power and available data. Classical scientific computing (accuracy vs. resources), uncertainty quantification (precision vs. resources) and stochastic modeling (precision vs. accuracy) can be associated with the edges of the triangle. Even along single edges, it is unknown how to determine optimal trade-offs in view of multiple optimization criteria. In the expectation of a more powerful yet also more heterogeneous hardware structure, we plan on tailoring and dynamically optimizing models and simulations in this triangle. The key issue will be the integration of information derived not only from experimental data but also from metadata, i.e., data about the simulations themselves.
Research questions
RQ 1 Accuracy vs. resources: How can we dynamically adapt towards specific accuracy targets, given the hardware, time and power constraints imposed by a heterogeneous, yet undefined, hardware landscape?
RQ 2 Precision vs. resources: How can we quantify uncertainties and dynamically ensure confidence in simulations given randomness in observational data, limitations of computational resources and incomplete models?
RQ 3 Precision vs. accuracy: How can we construct and dynamically adjust stochastic models with optimized complexity despite limited domain knowledge and limited availability of calibration data?
RQ 4 The triangle: How can we ultimately quantify and adaptively approach global minima for resource requirements given a prescribed total error (accuracy plus precision) target?
Ongoing projects
Completed projects
Completed associated projects
Project Network Coordinators
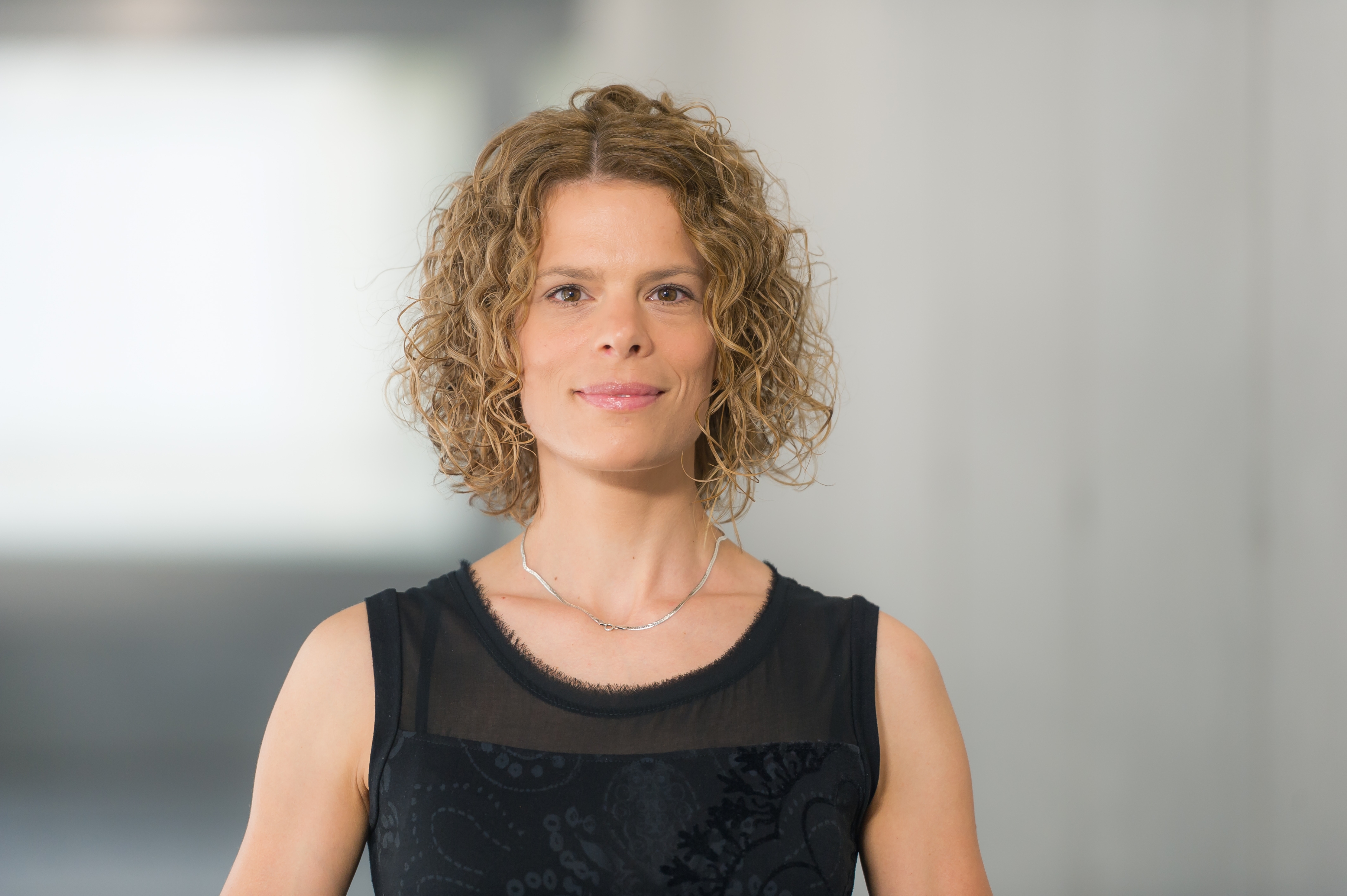
Andrea Barth
Prof. Dr.Computational Methods for Uncertainty Quantification
[Photo: SimTech/Max Kovalenko]
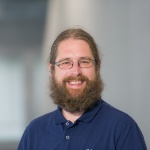
Dominik Göddeke
Prof. Dr. rer. nat.Computational Mathematics for Complex Simulations in Science and Engineering
[Photo: SimTech/Max Kovalenko]