Publications PN 4
2023
- A. Baier, D. Aspandi Latif, and S. Staab, “Supplements for ‘ReLiNet: Stable and Explainable Multistep Prediction with Recurrent Linear Parameter Varying Networks’".” 2023. doi: 10.18419/darus-3457.
- T. Holicki, J. Nicodemus, P. Schwerdtner, and B. Unger, “Energy matching in reduced passive and port-Hamiltonian systems.” 2023. doi: 10.48550/arXiv.2309.05778.
- R. Strässer, J. Berberich, and F. Allgöwer, “Control of bilinear systems using gain-scheduling: Stability and performance guarantees,” in 62nd IEEE Conference on Decision and Control (CDC), in 62nd IEEE Conference on Decision and Control (CDC). Singapore, Singapore, 2023, pp. 4674–4681. doi: 10.1109/CDC49753.2023.10384021.
- S. Schlor, R. Strässer, and F. Allgöwer, “Koopman interpretation and analysis of a public-key cryptosystem: Diffie-Hellman key exchange,” in Proceedings of the 22nd IFAC World Congress, in Proceedings of the 22nd IFAC World Congress. Yokohama, Japan, 2023, pp. 984–990. doi: 10.1016/j.ifacol.2023.10.1693.
- R. Strässer, J. Berberich, and F. Allgöwer, “Robust data-driven control for nonlinear systems using the Koopman operator,” in Proceedings of the 22nd IFAC World Congress, in Proceedings of the 22nd IFAC World Congress, vol. 56. 2023, pp. 2257–2262. doi: https://doi.org/10.1016/j.ifacol.2023.10.1190.
- O. V. Martynenko et al., “Development and verification of a physiologically motivated internal controller for the open-source extended Hill-type muscle model in LS-DYNA,” Biomechanics and Modeling in Mechanobiology, vol. 22, no. 6, Art. no. 6, 2023, doi: 10.1007/s10237-023-01748-9.
- T. Martin and F. Allgöwer, “Data-driven inference on optimal input-output properties of polynomial systems with focus on nonlinearity measures,” IEEE Transactions on Automatic Control, vol. 68, no. 5, Art. no. 5, 2023, doi: 10.1109/TAC.2022.3226652.
- T. Martin, T. B. Schön, and F. Allgöwer, “Guarantees for data-driven control of nonlinear systems using semidefinite programming: A survey,” Annual Reviews in Control, vol. 56, p. 100911, 2023, doi: 10.1016/j.arcontrol.2023.100911.
- D. Gramlich, T. Holicki, C. W. Scherer, and C. Ebenbauer, “A Structure Exploiting SDP Solver for Robust Controller Synthesis,” IEEE Control Syst. Lett., vol. 7, pp. 1831–1836, 2023, doi: 10.1109/LCSYS.2023.3277314.
- M. M. Morato, T. Holicki, and C. W. Scherer, “Stabilizing Model Predictive Control Synthesis using Integral Quadratic Constraints and Full-Block Multipliers,” International Journal of Robust and Nonlinear Control, 2023, doi: 10.1002/rnc.6952.
- T. Martin and F. Allgöwer, “Data-driven system analysis of nonlinear systems using polynomial approximation,” IEEE Trans. Automat. Control (early access), 2023, doi: 10.1109/TAC.2023.3321212.
- A. Kharitenko and C. Scherer, “Time-varying Zames–Falb multipliers for LTI Systems are superfluous,” Automatica, vol. 147, p. 110577, Jan. 2023, doi: 10.1016/j.automatica.2022.110577.
- C. D. Remy, Z. Brei, D. Bruder, J. Remy, K. Buffinton, and R. B. Gillespie, “The ‘Fluid Jacobian’: Modeling force-motion relationships in fluid-driven soft robots,” The International Journal of Robotics Research, Nov. 2023, doi: 10.1177/02783649231210592.
- T. Holicki and C. W. Scherer, “IQC based analysis and estimator design for discrete-time systems affected by impulsive uncertainties,” Nonlinear Analysis: Hybrid Systems, vol. 50, p. 101399, 2023, doi: 10.1016/j.nahs.2023.101399.
- A. Alanwar, A. Koch, F. Allgöwer, and F. H. Johansson, “Data-Driven Reachability Analysis from Noisy Data,” IEEE Transactions on Automatic Control, pp. 1–16, 2023, doi: 10.1109/TAC.2023.3257167.
2022
- T. Holicki, “A Complete Analysis and Design Framework for Linear Impulsive and Related Hybrid Systems,” University of Stuttgart, 2022. doi: 10.18419/opus-12158.
- D. Gramlich, C. W. Scherer, and C. Ebenbauer, “Robust Differential Dynamic Programming,” in 2022 IEEE 61st Conference on Decision and Control (CDC), in 2022 IEEE 61st Conference on Decision and Control (CDC). 2022. doi: 10.1109/cdc51059.2022.9992569.
- C. Fiedler, C. W. Scherer, and S. Trimpe, “Learning Functions and Uncertainty Sets Using Geometrically Constrained Kernel Regression,” in 61st IEEE Conf. Decision and Control, in 61st IEEE Conf. Decision and Control. IEEE, Dec. 2022. doi: 10.1109/cdc51059.2022.9993144.
- T. Martin and F. Allgöwer, “Determining dissipativity for nonlinear systems from noisy data using Taylor polynomial approximation,” in Proc. American Control Conf. (ACC), in Proc. American Control Conf. (ACC). Atlanta, GA, USA, 2022, pp. 1432–1437.
- J. Nicodemus, J. Kneifl, J. Fehr, and B. Unger, “Physics-informed Neural Networks-based Model Predictive Control for Multi-link Manipulators,” IFAC-PapersOnLine, vol. 55, no. 20, Art. no. 20, 2022, doi: 10.1016/j.ifacol.2022.09.117.
- D. Gramlich, C. Ebenbauer, and C. W. Scherer, “Synthesis of Accelerated Gradient Algorithms for Optimization and Saddle Point Problems using Lyapunov functions,” Systems & Control Letters, vol. 165, 2022, doi: 10.1016/j.sysconle.2022.105271.
- J. Berberich, C. W. Scherer, and F. Allgöwer, “Combining Prior Knowledge and Data for Robust Controller Design,” IEEE Transactions on Automatic Control, vol. 68, no. 8, Art. no. 8, 2022, doi: 10.1109/tac.2022.3209342.
- C. Scherer, “Dissipativity and Integral Quadratic Constraints, Tailored computational robustness tests for complex interconnections,” IEEE Control Systems Magazine, vol. 42, no. 3, Art. no. 3, 2022, [Online]. Available: https://arxiv.org/abs/2105.07401
- F. Kempter, L. Lantella, N. Stutzig, J. Fehr, and T. Siebert, “Role of Rotated Head Postures on Volunteer Kinematics and Muscle Activity in Braking Scenarios Performed on a Driving Simulator,” Annals of Biomedical Engineering, vol. 51, no. 4, Art. no. 4, 2022, doi: 10.1007/s10439-022-03087-9.
2021
- C. Fiedler, C. W. Scherer, and S. Trimpe, “Practical and Rigorous Uncertainty Bounds for Gaussian Process Regression,” in Proceedings of the AAAI Conference on Artificial Intelligence, in Proceedings of the AAAI Conference on Artificial Intelligence, vol. 35. 2021, pp. 7439–7447. [Online]. Available: https://ojs.aaai.org/index.php/AAAI/article/view/16912
- J. Veenman, C. W. Scherer, C. Ardura, S. Bennani, V. Preda, and B. Girouart, “IQClab: A new IQC based toolbox for robustness analysis and control design,” in IFAC-PapersOnline, in IFAC-PapersOnline, vol. 54. 2021, pp. 69--74. doi: 10.1016/j.ifacol.2021.08.583.
- C. Fiedler, C. W. Scherer, and S. Trimpe, “Learning-enhanced robust controller synthesis with rigorous statistical and control-theoretic guarantees,” in 60th IEEE Conference Decision and Control, in 60th IEEE Conference Decision and Control. 2021.
- R. Strässer, J. Berberich, and F. Allgöwer, “Data-Driven Control of Nonlinear Systems: Beyond Polynomial Dynamics,” in Proc. 60th IEEE Conf. Decision and Control (CDC), in Proc. 60th IEEE Conf. Decision and Control (CDC). Austin, TX, USA, 2021, pp. 4344–4351. doi: 10.1109/CDC45484.2021.9683211.
- A. Alanwar, A. Koch, F. Allgöwer, and K. H. Johansson, “Data-Driven Reachability Analysis Using Matrix Zonotopes,” in Proceedings of the 3rd Conference on Learning for Dynamics and Control, in Proceedings of the 3rd Conference on Learning for Dynamics and Control, vol. 144. 2021, pp. 163--175.
- N. Wieler, J. Berberich, A. Koch, and F. Allgöwer, “Data-Driven Controller Design via Finite-Horizon Dissipativity,” in Proceedings of the 3rd Conference on Learning for Dynamics and Control, in Proceedings of the 3rd Conference on Learning for Dynamics and Control, vol. 144. PMLR, 2021, pp. 287--298.
- S. Schlor, M. Hertneck, S. Wildhagen, and F. Allgöwer, “Multi-party computation enables secure polynomial control based solely on secret-sharing,” in Proc. 60th IEEE Conf. Decision and Control (CDC), in Proc. 60th IEEE Conf. Decision and Control (CDC). Austin, TX, USA, 2021, pp. 4882–4887. doi: 10.1109/CDC45484.2021.9683026.
- S. Michalowsky, C. Scherer, and C. Ebenbauer, “Robust and structure exploiting optimisation algorithms: An integral quadratic constraint approach,” International Journal of Control, vol. 94, no. 11, Art. no. 11, 2021, doi: 10.1080/00207179.2020.1745286.
- T. Holicki, C. W. Scherer, and S. Trimpe, “Controller Design via Experimental Exploration with Robustness Guarantees,” IEEE Control Systems Letters, vol. 5, no. 2, Art. no. 2, 2021, doi: 10.1109/LCSYS.2020.3004506.
- A. Koch, J. Berberich, and F. Allgöwer, “Provably robust verification of dissipativity properties from data,” IEEE Transactions on Automatic Control, vol. 67, no. 8, Art. no. 8, 2021, doi: 10.1109/TAC.2021.3116179.
- T. Holicki and C. W. Scherer, “Robust Gain-Scheduled Estimation with Dynamic D-Scalings,” EEE Transactions on Automatic Control, vol. 66, no. 11, Art. no. 11, 2021, doi: 10.1109/TAC.2021.3052751.
- A. Koch, J. M. Montenbruck, and F. Allgöwer, “Sampling Strategies for Data-Driven Inference of Input-Output System Properties,” IEEE Transactions On Automatic Control, vol. 66, pp. 1144–1159, 2021, doi: 10.1109/TAC.2020.2994894.
- A. Koch, J. Berberich, J. Köhler, and F. Allgöwer, “Determining optimal input–output properties: A data-driven approach,” Automatica, vol. 134, p. 109906, 2021, doi: https://doi.org/10.1016/j.automatica.2021.109906.
- T. Holicki and C. W. Scherer, “Revisiting and Generalizing the Dual Iteration for Static and Robust Output-Feedback Synthesis,” Int. J. Robust Nonlin., vol. 31, no. 11, Art. no. 11, 2021, doi: 10.1002/rnc.5547.
- T. Martin and F. Allgöwer, “Dissipativity verification with guarantees for polynomial systems from noisy input-state data,” IEEE Control Systems Letters, vol. 5, no. 4, Art. no. 4, 2021, doi: 10.1109/LCSYS.2020.3037842.
2020
- T. Martin, A. Koch, and F. Allgöwer, “Data-driven surrogate models for LTI systems via saddle-point dynamics,” in Proc. 21st IFAC World Congress, in Proc. 21st IFAC World Congress. Berlin, Germany, 2020, pp. 971–976. doi: 10.1016/j.ifacol.2020.12.1261.
- T. Martin and F. Allgöwer, “Iterative data-driven inference of nonlinearity measures via successive graph approximation,” in Proceedings 59th IEEE Conference Decision and Control (CDC), in Proceedings 59th IEEE Conference Decision and Control (CDC). Jeju, South Korea, 2020, pp. 4760–4765. doi: 10.1109/CDC42340.2020.9304285.
- M. Barreau, C. W. Scherer, F. Gouaisbaut, and A. Seuret, “Integral Quadratic Constraints on Linear Infinite-dimensional Systems for Robust Stability Analysis,” in IFAC-PapersOnline, in IFAC-PapersOnline, vol. 53. 2020, pp. 7752–7757. [Online]. Available: https://www.sciencedirect.com/science/article/pii/S2405896320321297
- D. Persson, A. Koch, and F. Allgöwer, “Probabilistic H2-norm estimation via Gaussian process system identification,” in Proceedings 21st IFAC World Congress, in Proceedings 21st IFAC World Congress. Berlin, Germany, 2020, pp. 431–436. doi: 10.1016/j.ifacol.2020.12.211.
- A. Koch, J. Berberich, and F. Allgöwer, “Verifying dissipativity properties from noise-corrupted input-state data,” in Proceedings 59th IEEE Conference on Decision and Control (CDC), in Proceedings 59th IEEE Conference on Decision and Control (CDC). Jeju, South Korea, 2020, pp. 616–621. doi: 10.1109/CDC42340.2020.9304380.
- D. F. B. Häufle, I. Wochner, D. Holzmüller, D. Drieß, M. Günther, and S. Schmitt, “Muscles reduce neuronal information load : quantification of control effort in biological vs. robotic pointing and walking,” Frontiers in Robotics and AI, vol. 7, p. 77, 2020, doi: 10.3389/frobt.2020.00077.
- C. A. Rösinger and C. W. Scherer, “A Flexible Synthesis Framework of Structured Controllers for Networked Systems,” IEEE Trans. Control Netw. Syst., vol. 7, no. 1, Art. no. 1, 2020, doi: 10.1109/TCNS.2019.2914411.
2019
- T. Holicki and C. W. Scherer, “A Homotopy Approach for Robust Output-Feedback Synthesis,” in Proc. 27th. Med. Conf. Control Autom., in Proc. 27th. Med. Conf. Control Autom. 2019, pp. 87–93. doi: 10.1109/MED.2019.8798536.
- G. Baggio, S. Zampieri, and C. W. Scherer, “Gramian Optimization with Input-Power Constraints,” in 58th IEEE Conf. Decision and Control, in 58th IEEE Conf. Decision and Control. 2019, pp. 5686–5691. doi: 10.1109/CDC40024.2019.9029169.
- A. Romer, J. Berberich, J. Köhler, and F. Allgöwer, “One-shot verification of dissipativity properties from input-output data,” IEEE Control Systems Letters, vol. 3, pp. 709–714, 2019, doi: 10.1109/LCSYS.2019.2917162.
- T. Holicki and C. W. Scherer, “Stability Analysis and Output-Feedback Synthesis of Hybrid Systems Affected by Piecewise Constant Parameters via Dynamic Resetting Scalings,” Nonlinear Analysis: Hybrid Systems, vol. 34, pp. 179–208, 2019, doi: https://doi.org/10.1016/j.nahs.2019.06.003.
2018
- A. Romer, J. M. Montenbruck, and F. Allgöwer, “Data-driven inference of conic relations via saddle-point dynamics,” in Proceedings 9th IFAC Symposium Robust Control Design (ROCOND), in Proceedings 9th IFAC Symposium Robust Control Design (ROCOND). Florianópolis, Brazil, 2018, pp. 586–591. doi: 10.1016/j.ifacol.2018.11.139.
Software PN 4
2023
- A. Baier, D. Aspandi Latif, and S. Staab, “Supplements for ‘ReLiNet: Stable and Explainable Multistep Prediction with Recurrent Linear Parameter Varying Networks’".” 2023. doi: 10.18419/darus-3457.
- F. Kempter, L. Lantella, N. Stutzig, J. C. Fehr, and T. Siebert, “Neck Reflex Behavior in Driving Simulator Experiments - Academic-Scale Simulator at ITM.” 2023. doi: 10.18419/darus-3000.
Data PN 4
2022
- A. Baier and S. Staab, “A Simulated 4-DOF Ship Motion Dataset for System Identification under Environmental Disturbances.” 2022. doi: 10.18419/darus-2905.
Project Network Coordinators
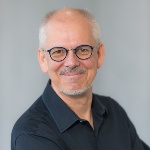
Frank Allgöwer
Prof. Dr.-Ing.Mechanical Engineering
[Photo: SimTech/Max Kovalenko]
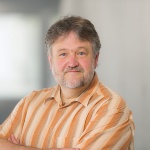
Peter Eberhard
Prof. Dr.-Ing. Prof. E.h.Engineering and Computational Mechanics
[Photo: SimTech/Max Kovalenko]