Biomarkers are biological characteristics that can be detected in blood or tissue. These can be genetic changes that occur in cancer cells. Biomarkers can therefore provide information about the specific characteristics of a tumor. They can also be used to select an individual therapy.
Despite considerable progress in prevention, diagnosis, and patient management, breast cancer is still the second most common cause of cancer death in women. The risk of breast cancer and the course of the disease can be predicted using certain methods. For example, using individual biomarkers or multi-gene expression tests. However, the latter are approved only for patients in certain risk groups.
This is why Dr. Markus Morrison, Professor and Head of the Institute of Cell Biology and Immunology at the University of Stuttgart, and his research assistant Dr. Cristiano Guttà, in collaboration with the Ludwigsburg-based company ProKanDo, which specializes in IT security and AI solutions, have developed a method that not only determines the risk for all patients using individual biomarkers or several high-risk genes but also takes into account a person’s entire genetic expression profile. Around 18,000 genes per person are included in the simulation. The researchers also generated artificial data sets in order to improve the general validity and reliability of the prognoses.
Virtual patients improve statistical significance
Morrison and Guttà used two large data sets of breast cancer patients as a basis. These contained both gene expression information and clinical data (e.g., how long a patient lived after diagnosis).
With multi-gene expression tests, several genes can be analyzed simultaneously. The tests are used to characterize complex biological relationships and diagnose diseases as well as to predict the course of a disease or the effectiveness of treatments.
However, for statistical predictions, it is difficult to derive good prognoses for the general public because of the large number of these genes per person (18,000) and the relatively small group of patients (around 1,400). They have therefore artificially enlarged the data sets with the help of AI. “The patterns that are hidden in this patient data and which could be related to the course of the disease cannot be identified using conventional statistical methods,” explains Morrison. “That’s why in our concept study we created virtual patients in silico – in other words, with the help of computers.” The more data there is, the more likely possible patterns that indicate a positive or negative prognosis can be identified.
A generative adversarial network (GAN) is a machine learning model. It consists of two opposing neural networks that compete with each other. One network, the generator, produces the data; the other network, the discriminator or verifier, determines whether the data is genuine or not. The data generated in this way is as authentic as possible.
In order to create these virtual patients, Morrison and Guttà, together with Christoph Morhard from ProKanDo, used a generative adversarial network (GAN) model from machine learning. This is used to generate images. “The deep learning algorithm learned how to generate images of genetic information that are so deceptively real that it was no longer possible to distinguish the data of the virtual patients from the data of the real breast cancer patients,” explains Guttà, who carried out this study as part of his doctoral thesis. The AI was trained with the data sets of real patients and then learned to generate even more artificial breast cancer data from them. “This, in turn, enabled the AI to learn from this larger data set and better distinguish between patients with a positive prognosis and patients with a negative prognosis,” adds Guttà. In this case, negative means that the cancer returns or progresses and the patient dies; positive means that they survive.
Recognizing patterns in genetic information using image recognition
“Our system, which is called T-GAN-D, uses deep learning algorithms originally designed for image analysis. This allows us to differentiate between breast cancer patients at high and low risk,” says Morrison. For this purpose, the genetic information is converted into images in which each pixel and the spatial values of these pixels in relation to each other represent the initial information. These images are then used as input for a classifier, which categorizes the patients into the respective risk category after it has been trained. “The results show that our system is on a par with established prognostic markers in the data sets investigated,” says Morrison. “Both the conversion of genetic information into images and the use of artificial data sets are promising approaches that could be a springboard for more individualized prognoses – also in combination with clinically established prognostic markers.”
A classifier is an algorithm used to categorize data into different categories. It analyzes characteristics of the data pattern and then makes decisions about the assignment to a specific class or category. Classifiers are often used in machine learning and data analysis in order to identify patterns in data and make predictions.
However, it is not yet easy to say exactly which genes are responsible for whether the prognosis is positive or negative. Morrison and Guttà therefore want to continue researching this method. “Together with clinical partners, it would be worthwhile to further improve our method. For example, by focusing on the most important genes for disease progression according to biomedical knowledge. This would allow us to test whether the prognoses are even more reliable,” says Guttà. This would allow future treatment strategies to be adapted at an early stage.
Because if you already know there is a high risk of relapse, the examination appointments could be scheduled more frequently. Alternatively, additional treatment measures that reduce the risk of relapse but which might not have been initiated without a reliable prognosis could be implemented at an earlier stage. If the prognosis is positive, patients could be spared stressful treatments.
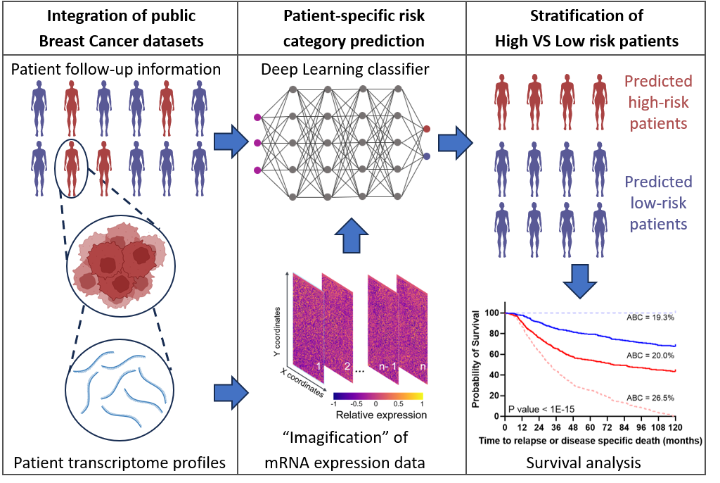
Should the algorithm continue to learn?
“We also have to consider whether we should leave the algorithm, which is now trained in this way, at this level of training or allow it to continue learning,” says Morrison. This is because more and better data sets are becoming publicly available, and new patients are continually being added. However, as soon as the algorithm continues to learn, it is difficult from a clinical-diagnostic point of view because the tests for diagnoses and prognoses are well established (i.e., they are precisely characterized, have a certain performance, which is also stored, and are carried out identically everywhere). “But in this case, it could lead to the model continually evolving. And for normal clinical practice and approval procedures, it is not yet clearly regulated how this should be handled,” says Morrison.
Another problem is that the raw data does not have a common international format because different laboratories use different methods and/or different machines. Nevertheless, the two scientists are pleased to have created a promising approach with their trained GAN discriminator (T-GAN-D), which could play a new and important role in improving the prognosis of cancer.
Manuela Mild | SimTech Science Communication
The study was conducted in collaboration with the Ludwigsburg-based company ProKanDo GmbH, which specializes in IT security and AI solutions.
Read more
Guttà, Cristiano & Morhard, Christoph & Rehm, Markus. (2023). Applying a GAN-based classifier to improve transcriptome-based prognostication in breast cancer. PLoS computational biology. https://doi.org/10.1371/journal.pcbi.1011035
About the scientists
Cristiano Guttà who comes from Italy, studied biology with a focus on cell and molecular biology, and completed his Master’s degree in a bioinformatics laboratory. At the Institute of Physics at the University of Bari, he obtained a second Master’s degree, which focused on the development of digital infrastructures. This led him to programming and IT and finally to Markus Morrison at the University of Stuttgart, where he completed his doctorate in a SimTech project focusing on data analysis and AI. He now conducts research at the Institute of Cell Biology and Immunology.
Markus Morrison is a Professor and Head of the Institute of Cell Biology and Immunology at the University of Stuttgart. He studied biology with a focus on biophysics and then became interested in cell biology. He worked in medical faculties, mostly abroad, for around 15 years. Since joining the Institute of Cell Biology and Immunology as a professor in 2016, he has been focusing more on basic research. He continues to conduct translational research (i.e., research that is clinically relevant and has a clinical perspective) with cooperation partners as well as in a project in SimTech.